- Docente: Giuliano Galimberti
- Crediti formativi: 8
- SSD: SECS-S/01
- Lingua di insegnamento: Inglese
- Modalità didattica: Convenzionale - Lezioni in presenza
- Campus: Bologna
- Corso: Laurea in Scienze statistiche (cod. 8873)
-
dal 17/09/2024 al 12/12/2024
Conoscenze e abilità da conseguire
By the end of the course the student should know the basic theory of normal linear models and generalized linear models. In particular the student should be able: - to define a statistical model - to formulate the normal linear model, estimate its parameters and test their significance - to use the variable selection procedures - to define a generalized linear model, by combining a random component with a linear predictor with a proper link function - to estimate and test the significance of the parameter of a generalized linear model - to evaluate the goodness of fit of a model and to detect violations of model assumptions
Contenuti
- Basic framework for linear models
- model specification and assumptions;
- parameter estimation: least squares and maximum likelihood methods;
- coefficient of determination R2: definition and properties;
- finite and asymptotic properties of the estimators;
- hypothesis testing on regression coefficients.
- Regression diagnostics
- residuals: definitions and properties;
- Influential observations and leverage points;
- Multicollinearity.
- Model selection
- effects of model mispecification;
- stepwise methods;
- best subset selection.
- Inclusion of qualitative regressors
- dummy variable coding;
- interactions between regressors.
- Some special cases
- one-way ANOVA;
- two-way ANOVA.
- Generalized linear models
- general definition: linear predictor, link function, random component;
- maximum likelihood estimation;
- hypothesis testing on model parameters.
Testi/Bibliografia
Recommended readings (a detailed list of selected chapters and sections in available on virtuale.unibo.it):
Kutner, M. H., Nachsteim, C. J., Neter, J., Li, W. (2005). Applied Linear Statistical Models (5th edition). McGraw-Hill.
Montgomery, D. C., Peck, E. A., Vining, G.G. (2021) Introduction to linear regression analysis. Sixth edition. Wiley.
Handouts provided by the teacher.
Other readings:
Brown, J. D. (2015). linear Models in Matrix Form. Springer.
Fox J. (2016). Applied Regression Analysis and Generalized Linear Models (3rd edition). Sage.
Weisberg S. (2005). Applied Linear Regression. Wiley, third edition.
Metodi didattici
Class lectures
Tutorial sessions in computer lab or using personal laptops in classroom
As concerns the teaching methods of this course unit, all students must attend Module 1, 2 on Health and Safety online
Modalità di verifica e valutazione dell'apprendimento
The exam will test the qualifications of each student on both a theoretical and a practical level.
The exam is composed of two parts that have to be taken during the same exam sitting.
The first mandatory part consists in a (possibly computer-based) quiz containing a set of multiple-choice and open-answer questions about the models presented during the course. These questions focus both on theoretical properties and on R instructions or output produced using the software R. As far as the multiple-choice questions are concerned, correct answers are marked with 1 point, wrong answers are marked -0.20 points and missing answers 0 points. Each open-answer question receives a mark ranging between 0 and 2, depending on the correctness of the answer and the appropriateness of the terminology. The number of multiple-choice and open-answer questions may vary from sitting to sitting, holding fixed the maximum mark to 24. Students will have 60 minutes to complete this quiz. Consulting textbooks or personal notes during the written exam is not allowed.
As far as the first sitting is concerned, students have the option of splitting the mandatory quiz exam into two partial exams, each lasting 30 minutes and with a maximum mark fixed at 12. The first partial quiz takes place after the first 5 weeks and is focused on the topics covered during the first part of the course. The second partial quiz is scheluled after the end of the course, and covers the topics addressed during the second part of the course. Students must take both partial quiz exams. In particular, in order to register for the second partial quiz, a student must have taken the first partial quiz. Furthermore, students taking the first partial quiz are not allowed to take the total quiz exam during the first sitting after the end of the course.
The second mandatory part is a computer-based practical exam. The practical exam assesses the ability of a student in uisng R to solve a practical problem. Students will be asked to write an R script and to report results obtained using that script. Students will reveive a mark ranging between 0 and 7, depending on the correctness of the answer and the appropriateness and correctness of the corresponding R script. Students will have 30 minutes to complete this second mandatory part. Consulting textbooks or personal notes during the practical exam is allowed.
The final mark is given by the sum of the marks obtained in the practical and in the written exam. Non-integer final marks are rounded down to the next small integer. Final marks larger that 30 are rounded down to 30. Final marks equal to 31 are considered 30 cum laude.
In case of failure or rejection of the overall mark, students must repeat the whole exam in one of the following sittings. In particular, students are allowed to reject a positive mark and retake the exam at least once but no more than twice.
Orario di ricevimento
Consulta il sito web di Giuliano Galimberti
SDGs
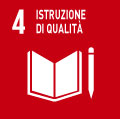
L'insegnamento contribuisce al perseguimento degli Obiettivi di Sviluppo Sostenibile dell'Agenda 2030 dell'ONU.