- Docente: Margherita Porcelli
- Crediti formativi: 6
- SSD: MAT/08
- Lingua di insegnamento: Inglese
- Moduli: Margherita Porcelli (Modulo 1) Davide Palitta (Modulo 2)
- Modalità didattica: Convenzionale - Lezioni in presenza (Modulo 1) Convenzionale - Lezioni in presenza (Modulo 2)
- Campus: Bologna
- Corso: Laurea Magistrale in Matematica (cod. 5827)
-
Orario delle lezioni (Modulo 1)
dal 29/03/2023 al 26/05/2023
-
Orario delle lezioni (Modulo 2)
dal 22/02/2023 al 24/03/2023
Conoscenze e abilità da conseguire
At the end of the course, students have theoretical and computational knowledge on matrix and tensor techniques for analysing large amounts of data. In particular, students are able to examine large samples of discrete data and extract interpretable information of relevance in image and data processing, in medical and scientific applications, and in social and security sciences.
Contenuti
The course presents fundamental matrix and tensor techniques commonly employed in Data Mining methods, together with optimization strategies designed to handle constrained problems typically arising in data science.
Mathematical tools will be developed for the following topics:
- Compression and sparse approximation strategies (PCA, non-negative factorizations, OMP, etc)
- Dictionary learning
- Matrix completion
Part I. The course presents fundamental matrix and tensor techniques commonly employed in large Data analysis methods, typically arising in data science. These will also serve as preparatory material for Part 2 on optimization strategies for the analysis of big data.
Part 1
- Vector and matrix norms (including sparsity promoting)
- Linear regression and Least squares
- Eigenvalues, SVD, pseudoinverse
- Reduction and low rank representation
- Sparse representation with l_0-norm
- Dictionary Learning: the Orthogonal Matching Pursuit (OMP) algorithm
- Principal Component Analysis (PCA) and Factor analysis
- Tensors
- Dealing with tensors and various representations
- HOSVD, Tensor OMP, Dictionary Learning with tensors (Alternating Least-Squares)
Part 2
Numerical optimization
- Basic concepts of unconstrained optimization and optimality conditions.
- Algorithms for unconstrained smooth optimization (first and second order methods, line-search strategies, convergence and complexity analysis)
- Algorithms for convex constrained optimization (projected gradient methods,convergence and complexity analysis)
- Algorithms for composite optimization (proximal gradient methods, convergence and complexity analysis)
Matrix and tensor optimization
- The Matrix Completion problem (problem analysis and proximal gradient approaches)
- Applications: recommender systems, data predictions
- The Dictionary Learning problem (problem analysis, greedy algorithms and proximal gradient approaches)
- Applications: object and text classification, face recognition
Computational experience in Matlab/Octave on realistic data will accompany the lectures.
Testi/Bibliografia
- Nocedal, Jorge, and Stephen Wright. Numerical optimization. Springer Science & Business Media, 2006.
- Beck, Amir. First-order methods in optimization. Society for Industrial and Applied Mathematics, 2017.
- Gillis, Nicolas. Nonnegative Matrix Factorization. SIAM, 2020.
- Dumitrescu, Bogdan, and Paul Irofti. Dictionary learning algorithms and applications. Springer, 2018.
Other textbooks, recent scientific articles and case studies from real world applications will be made available during the course.
Metodi didattici
Blackboard, slides and computer lab sessions.
Modalità di verifica e valutazione dell'apprendimento
Oral presentation of a takehome project (with slides)
Strumenti a supporto della didattica
Slides made available as pdf file on the course webpage. Use of Matlab computational environment, and various toolboxes.
Orario di ricevimento
Consulta il sito web di Margherita Porcelli
Consulta il sito web di Davide Palitta
SDGs
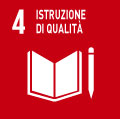
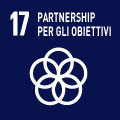
L'insegnamento contribuisce al perseguimento degli Obiettivi di Sviluppo Sostenibile dell'Agenda 2030 dell'ONU.