- Docente: Margherita Fort
- Crediti formativi: 6
- SSD: SECS-P/05
- Lingua di insegnamento: Inglese
- Modalità didattica: Convenzionale - Lezioni in presenza
- Campus: Bologna
- Corso: Laurea Magistrale in Economics (cod. 8408)
Conoscenze e abilità da conseguire
The course illustrates the most recent identification strategies for the quantitative assessment of causal effects using observational data by referring to micro-econometric applications. It will cover matching and difference-in-differences strategies, and quasi-experimental approaches to identification. At the end of the class, student will be able: - to critically understand the application of these tools in the recent empirical economic literature; - to apply these approaches to design his/her own program evaluation.
Contenuti
Il corso illustra le strategie empiriche adatte a ricerca applicata in campo della micro-econometria. L'obiettivo principale del corso è quello di fornire una panoramica degli strumenti statistici per l'analisi controfattuale. Il corso illustra le strategie di identificazione, la stima e altre questioni correlate (ad esempio la validità interna ed esterna) rilevanti per la valutazione degli effetti causali (o degli effetti di trattamento equivalentemente)utilizzando dati osservazionali. In particolare coprirà un'introduzione a una serie di strumenti di base: matching e difference in differences, variabili strumentali ed esperimenti quasi -naturali. Inoltre, toccherà brevemente approcci che consentono effetti di trattamento eterogenei e non presuppongono una struttura addittiva.
Il modello di effetti fissi ed effetti casuali sono trattati in altri corsi offerti nel percorso formativo e non saranno discussi in dettaglio. Toccheremo i modelli di effetti fissi, perché sono legati all'approccio difference in differences all'identificazione (e questo è un pre-requisito per discutere l'approccio di synthetic control methods e difference in discontinuities).
Verranno illustrate le strategie variabili strumentali in quanto esse sono necessarie per discutere la strategia del Fuzzy Regression Discontinuity Design. Si prega di notare che, rispetto ai corsi di econometria standard, discuteremo questioni avanzate relative a IV (interpretazione LATE, identificazione di momenti oltre le medie, validità esterna).
Parleremo di esperimenti (Randomized Control Trials) perché rappresentano il punto di riferimento dei metodi non sperimentali. I metodi sperimentali sono illustrati e discussi in dettaglio in altri corsi a livello di laurea e di laurea.
Le caratteristiche di ogni particolare strumento econometrico saranno illustrate sia dal punto di vista teorico che pratico, spesso attraverso la discussione di applicazioni empiriche.
L'accento sarà posto sull'attuazione pratica di ciascun approccio.
Argomenti
- Fundamentals of Impact Evaluation (circa 9 ore incluse le sessioni pratiche)
- The Fundamental Problem of Causal Inference
- Potential Outcomes Framework
- Basic Approaches to Identification:Randomized Trials
- Basic Approaches to Identification:Selection on Observables
- Basic Approaches to Identification: Panel Data/Repeated Cross Section Strategies: Difference-in-Differences
- Synthetic Control Methods
- Quasi-Experiments: IV (circa 15 ore incluse le sessioni pratiche)
- Instrumental Variables: Identification, Estimation, Falsification Checks (Placebo), Interpretation (LATE), External Validity; Weak Instruments; (Fuzzy Difference-in-Differences)
- Quantile Regression (QR) for Impact Evaluation: QR with exogenous regressors; IV strategies with quantile regression: The LATE-QTE model (Abadie et al. 2002); Causal Chain Model (Chesher, 2003); The IV-QTE Model (Chernozhucov et al. 2005)
- Quasi-Experiments: Jumps and Kinks (circa 6 ore incluse le sessioni pratiche)
- Regression Discontinuity Design: Sharp and Fuzzy Designs, Identification, Estimation, Falsification Checks
- Multiple Cutoffs, Multiple Running Variables (eg. Geographic RDD)
- External Validity: Extrapolating Away from the Cutoff
- Regression Kink Designs: Overview (identification, estimation, falsification checks)
Testi/Bibliografia
Le lezioni riguarderanno materiale ripreso dalle fonti elencate in seguito. Gli articoli relativi alle applicazioni empiriche si riferiranno alla letteratura più recente e la lista verrà fornita all'inizio del corso.
LIBRI
Mostly Harmless Econometrics, Angrist and Pischke
Mastering 'Metrics: The Path from Cause to Effect, Angrist and Pischke
ARTICOLI
TOPIC 1: FUNDAMENTALS OF IMPACT EVALUATION
Potential outcomes, experimental benchmark, selection on observed an unobserved variables
• Abadie, A. and Cattaneo, M. (2017) Econometric Methods for Program Evaluation mimeo available at: https://economics.mit.edu/files/13924
• Holland, Paul W (1986) Statistics and Causal Inference, Journal of the American Statistical Association 81 (396): pp. 945-970, with discussion
Difference-in-Differences
• Athey and Imbens (2006) Identification and Estimation in Nonlinear Difference-in-Differences Models, Econometrica 74(2) pp.431-497
• Betrand et al. (2004) How Much Should We Trust Difference-in-Differences Estimates?, Quarterly Journal of Economics 119, pp. 245-279
• Card, D and Krueger, A. (1994) Minimum Wages and Employment: A Case Study of the Fast-Food Industry in New Jersey and Pennsylvania, American Economic Review 84(4), pp. 772-793
Computing Standard Errors
• Abadie, A. and Athey, S. and Imbens, G. (2017) When Should You Adjust Standard Errors for Clustering? WP October 2017
Propensity Score Matching
• Dehejia, R.H. and Wahba, S. (1999) Causal Effects in Non-Experimental Studies: Re-evaluating the Evaluation of Training Programs, Journal of the American Statistical Association 94 (448) pp. 1053-1062
• Dehejia, R.H. and Wahba, S. (2002) Propensity Score-Matching Methods for Nonexperimental Causal Studies, Review of Economics and Statistics, 84(1), pp. 151-161
• Dehejia, R.H. and Wahba, S. (2005) Practical Propensity Score Matching. A Reply to Smith and Todd, Journal of Econometrics, 125, pp. 355-364.
• Rosenbaum, P.R. and Rubin, D.B. (1983) The Central Role of the Propensity Score in Observational Studies for Causal Effects, Biometrika 70(1), pp.41-55
• Rosenbaum, P.R. and Rubin, D.B. (1984) Reducing Bias in Observational Studies using Subclassification on the Propensity Score, Journal of the American Statistical Association 79 (387), pp. 147-156
• Smith, J. and Todd, P. (2005a) Does Matching Overcome Lalonde’s critique of Non-Experimental Estimators? Journal of Econometrics 125, pp. 305-353
• Smith, J. and Todd, P. (2005b) Rejonder Journal of Econometrics 125, pp. 365-375
Synthetic Control Methods
• Abadie, A. and Diamond, A. and Hainmueller, J. (2015) Comparative Politics and the Synthetic Control Method, American Journal of Political Science, 59(2), pp. 495-510
• Abadie, A. and Diamond, A. and Hainmueller, J. (2010) Synthetic Control Methods for Comparative Case Studies: Estimating the Effect of California’s Tobacco Control Program, Journal of the American Statistical Association, 105(490), pp. 493-505.
394-410 TOPIC 2: QUASI-EXPERIMENTS: IV
Instrumental Variables
• Angrist, J., Imbens, G. and Rubin, D. (1996) Identification of Causal Effects Using Instrumental Variables, Journal of the American Statistical Association, 91 (434) pp. 444-455, with discussion
• Angrist, J. and Graddy, K. and Imbens G. (2000) The Interpretation of Instrumental Variables Estimators in Simultaneous Equations Models with and Application to the Demand For Fish, Review of Economic Studies, 67 pp. 499-527
• Angrist, J. (2004) Treatment Effect Heterogeneity in Theory and Practice, The Economic Journal, 114 (494) p.C52-C83
• Angrist,and Fernandez-Val (2013) ExtrapoLATE-ing: External Validity and Overidentification in the LATE Framework, in Advances in Econometric Theory and Applications, 10th World Congress, Volume III
• Imbens, G. and Rubin, D. (1997) Estimating the Outcome Distribution for Compliers in Instrumental Variables Models, Review of Economic Studies, 64, pp. 555-574
• Bound, J. and Jaeger, D. and Baker, R. (1995) Problems with Instrumental Variables Estimation when the Correlation Between the Instruments and the Endogenous Variables is Weak, Journal of the American Statistical Association 90, 443-450
• Staiger and Stock (1997) Instrumental Variable Regressions with Weak Instruments, Econometrica 65 (3), pp. 557-586
• Yogo, M., Wright, J, and Stock (2002) A Survey of Weak Instruments and Weak Identification in Generalized Method of Moments, Journal of Business and Economic Statistics 20, pp. 518-529
Quantile regression
• Abadie, A. et al. (2002) Instrumental Variable Estimates of the Effect of Subsidized Training on the Quantiles of Trainee Earnings, Econometrica, Vol. 70 (1), pp. 91-117.
• Bitler, M. et al. (2006 What Mean Impacts Miss: Distributional Effects of Welfare Reforms Experiments, American Economic Review, 96 (4) pp. 988-1012
• Chernozhucov, V. et al. (2004) The Effects of 401(K) Participation on the Wealth Distribution: An Instrumental Quantile Regression Analysis, The Review of Economics and Statistics, Vol. 86 (3), pp. 735-751.
• Chernozhucov, V. et al. (2005) An IV Model of Quantile Treatment Effects, Econometrica, Vol. 73 (1), pp. 245-261.
• Chernozhucov, V. et al. (2006) Instrumental Quantile Regression Inference for Structural and Treatment Effect Models, Journal of Econometrics
• Chesher, A. (2003) Identification in Nonseparable Models, Econometrica, Vol. 71, pp. 1405-1441
• Heckman, J.J. and Smith, J. and Clements, N. (1997) Making the Most Out of Programme Evaluations and Social Experiments: Accounting for Heterogeneity in Programme Impacts, Review of Economic Studies, 64 (4), pp. 487-535
• Imbens, G. and Rubin, D. (1997) Estimating the Outcome Distribution for Compliers in Instrumental Variables Models, Review of Economic Studies, 64, pp. 555-574
• Koenker, R. and Hallock, K.F. (2002) Quantile Regression, Journal of Economic Perspectives 15(4) pp. 143-156
• Koenker, R. (2005) Quantile Regression, Cambridge University Press, Econometric Society Monograph 38
• Ma, L. et al (2006) Quantile Regression Methods for Recursive Structural Equation Models, Journal of Econometrics, 134(2), pp. 471-506
TOPIC 3 QUASI-EXPERIMENTS: JUMPS AND KINKS
Regression Discontinuity Design
• Angrist and Lavy (1999) Using the Maimonides Rule to Estimate the Effect of Class Size on Scholastic Achievement, Quarterly Journal of Economics
• Cook (2008) Waiting for Life to Arrive: A History of the Regression-Discontinuity Design in Psychology, Statistics and Economics, Journal of Econometrics 142, pp.636-654
• Hahn, J. and Todd, P. and Van der Klaauw, W. (2001) Identification and Estimation of Treatment Effects with a Regression Discontinuity Design, Econometrica 69 (1)
• Imbens, G. and Lemieux, T. (2008) Regression Discontinuity Designs: A Guide to Practice, Journal of Econometrics 142 (2), pp. 615-635 and papers on the same journal in the special issue The Regression Discontinuity Design: Theory and Applications, Journal of Econometrics 142(2), pp. 611-850
• Research work by Cattaneo and co-authors https://sites.google.com/site/matiasdcattaneo/
RD away from the cutoffs (external validity); multiple cutoffs; multiple running variables
• Angrist, J. and Rokkanen, M. (2015) Wanna Get Away? Regression Discontinuity Estimation of Exam School Effects Away from the Cutoff, Journal of the American Statistical Association
Metodi didattici
Lucidi, dispense di materiale didattico per il laboratorio, esercitazioni in laboratorio informatico, test di autovalutazione on-line tramite la piattaforma e-learning di Ateneo
Le lezioni riguarderanno la presentazione di argomenti teorici ed applicati relativi ai vari strumenti econometrici. Le applicazioni saranno illustrate in aula e successivamente riprese nelle lezioni pratiche in laboratorio informatico usando STATA.
Per rispondere alla necessità offrire il corso online, per rendere l'esperienza più interattiva verranno introdotti -in via sperimentale - elementi di didattica "innovativa" (peer instruction; vedere link con riferimento bibliografico in inglese) utilizzando strumenti adeguati come software disponibile gratuitamente in rete come Pingo (https://pingo.coactum.de/)
Gli studenti non devono installare alcuno di questi softwares prima della lezione ma devono poter utilizzare uno strumento (cellulare o laptop) che permetta di accedere ad internet durante la lezione in cui si seguirà l'approccio della peer education.
Questo approccio richiede un discreto investimento da parte degli studenti poichè è necessario che essi studino del materiale dal libro di testo (in totale autonomia) prima di partecipare alla lezione.
Modalità di verifica e valutazione dell'apprendimento
A causa dell'emergenza sanitaria legata a Covid-19, per la sessione di gennaio e febbraio 2021, l'esame del corso si svolgerà con modalità online.
La valutazione sarà basata su
- replication study (70%; max 23 su 33 punti)
Dovrà essere consegnato: a) il codice STATA per replicare le analisi di un paper pubblicato di recente che usa gli strumenti di analisi empirica presentati nel corso; b) un Fact checking report, che riassume i risultati dell'analisi replicata (standard illustrato agli studenti a lezione); c) lucidi di una presentazione che estende lo studio originario (ad esempio: stessa analisi su dati più recenti/riferiti ad altro paese; analisi differente sugli stessi dati)
- partecipazione durante le lezioni (20%; max 7 su 33 punti)
- risposta alle domande generali sul programma del corso durante la presentazione orale dei risultati del replication study (10% max 4 su 33 punti)
Strumenti a supporto della didattica
Lucidi, materiale didattico (codici di programmi per analisi dati) , pratica in laboratorio.
I test on-line di autovalutazione saranno resi disponibili attraverso la piattaforma di e-learning https://elearning-cds.unibo.it/
Le lezioni prevedono la presentazione di questioni teoriche e applicate dei vari metodi econometrici. Le applicazioni vengono discusse in classe e replicate durante la sessione di laboratorio del computer utilizzando STATA.
Software STATA: disponibile per gli studenti del Dipartimento di Economia (licenza CAMPUS) e presso il Laboratorio Informatico della Scuola di Economia e Gestione.
Link ad altre eventuali informazioni
https://www.kuleuven.be/english/education/teaching-tips/activating-students/peer-instruction)
Orario di ricevimento
Consulta il sito web di Margherita Fort
SDGs
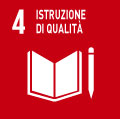
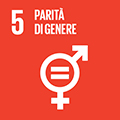
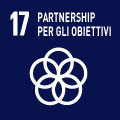
L'insegnamento contribuisce al perseguimento degli Obiettivi di Sviluppo Sostenibile dell'Agenda 2030 dell'ONU.