- Docente: Daniela Cocchi
- Crediti formativi: 6
- SSD: SECS-S/01
- Lingua di insegnamento: Inglese
- Modalità didattica: Convenzionale - Lezioni in presenza
- Campus: Bologna
-
Corso:
Laurea Magistrale in
Statistical Sciences (cod. 9222)
Valido anche per Laurea Magistrale in Statistical Sciences (cod. 9222)
Conoscenze e abilità da conseguire
By the end of the course the student knows the basis of Bayesian inference and has the tools for addressing the problems of parametric estimation, predictive inference and hypothesis testing according to the Bayesian viewpoint. In particular, the student is able to face the theoretical problems above and to use statistical software for estimating Bayesian models.
Contenuti
Comparison between classical and Bayesian framework. Open problems in the classical statistical models.
Bayes theorem for events and random variables. Revision of a prior via an experiment.
Bayes inference for events with discrete priors. Odds ratios for couples of events.
Posterior and predictive distributions for a Bernoulli likelihood and discrete and continuous prior distribution. The Beta distribution. Bayesian inference for the normal-normal case with known variance.
Sufficient statistics in Bayesian inference. Natural conjugate distributions.
One parameter exponential family.
Gamma Poisson model.
Gamma and Chi-square distribution.
Inference on the variance of the normal model with known mean.
Inference on the precision of the normal model with known mean.
Two parameters exponential family.
Non informative priors, reference priors.
Alternative formulation for the ignorance on the parameter of the Binomial distribution. Improper priors and Jeffrey's rule. Parameterization of the negative binomial distribution. Exchangeability and hierarchical models. Interval estimation: credibility intervals. Hypothesis testing.
Introduction to a computer code for Bayesian analysis.
Testi/Bibliografia
Lee P.M. (2012) Bayesian Statistics: An Introduction, 4th Edition, Wiley
Hoff P. (2009) A First Course in Bayesian Statistical Methods, Springer
Further Bibliographical references will be given at the beginning of the course
Metodi didattici
The course is made of lectures and computer sessions
Modalità di verifica e valutazione dell'apprendimento
The final test (2 hours) will consist of a computer session and a set of questions. The test (2 hours) takes place in one of the computer rooms. The computer session is be mainly devoted to the construction of a model to solve and assess via the software illustrated during the course. The questions focus on the theory and technical points illustrated during the course. After the correction, each candidate will briefly discuss her/his test.
The online test will be performed via “Esami on Line” (EOL). Zoom will be the platform for identification and monitoring.
Strumenti a supporto della didattica
Notes prepared by the teacher will be available.
The course is completed with computer session introducing a software for Bayesian analysis (Stan)
Orario di ricevimento
Consulta il sito web di Daniela Cocchi
SDGs
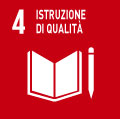
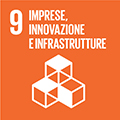
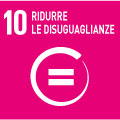
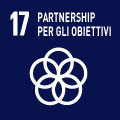
L'insegnamento contribuisce al perseguimento degli Obiettivi di Sviluppo Sostenibile dell'Agenda 2030 dell'ONU.