- Docente: Monica Palmirani
- Credits: 6
- SSD: IUS/20
- Language: English
- Moduli: Monica Palmirani (Modulo 1) Salvatore Sapienza (Modulo 2)
- Teaching Mode: Traditional lectures (Modulo 1) Traditional lectures (Modulo 2)
- Campus: Bologna
-
Corso:
Second cycle degree programme (LM) in
Legal Studies (cod. 9062)
Also valid for Single cycle degree programme (LMCU) in Law (cod. 9232)
-
from Sep 25, 2023 to Oct 20, 2023
-
from Oct 20, 2023 to Nov 13, 2023
Learning outcomes
The course is included in the Jean Monnet Module programme LEDS4XAIL [https://site.unibo.it/leds4xail] . It wants to cope with the following goals:
1. to provide the knowledge of the technical and legal definitions related to the Artificial Intelligence, data analytics, big data, open data, open government data and connected policies;2. to analyse the normative framework related to the AI Act and Data Governance (GAIA-X, EU regulation, GDPR, FOIA, PSI II, Licenses, Copyright Directive, DGA,DA, DSA);
3. to familiarize with some technical instruments for AI and data analytics as R, KNIME, Phyton for understanding the main principles;
4. to design an AI and data science project with legal data with an interdisciplinary methodology;
5. to analyse data science results under the quantitative and qualitative points of view;
6. to evaluate the explicability of the AI results (XAI and YAI);
7. to make an ethics evaluation of the AI models and Data Analytics methods in order to discover biases or other issues that could affect the human rights.
Course contents
At the end of the course, the student has the knowledge, competencies and skills to read and understand a quantitative and qualitative analysis of data manipulations with NLP and AI from activities related to the world of law (eg, judgments, justice statistics, legislation, administrative acts) in order to improve their legal profession. He/she is able to understand the data sources, to evaluate their completeness and consistency, as well as the quality in order to create an autonomous and argued opinion. He/she can analyze datasets in the light of data regulation (protection of personal data, licenses, competition, public law) and identify the legal issues to be addressed (anonymization, pseudo-anonymization, encryption).
Finally the data are manipulated using artificial intelligence techniques (e.g., Machine Learning, Generative AI, NLP), applied to the legal context that makes use of big data. In this way the student can understand how the datasets contribute to the final outcome of AI in order to provide a reasonable explanation of the conclusions. The student is encouraged to discover any cognitive biases introduced in the data and AI that could cause discrimination or in general regulatory violations and ethical criticalities.
Readings/Bibliography
Ashley, Kevin D. Artificial Intelligence and Legal Analytics. Cambridge University Press, 2017.
Livermore, Michael, and Daniel Rockmore, eds. Law as Data: Computation, Text, and the Future of Legal Analysis. SFI Press, 2019.
https://www.datascienceforlawyers.org/
http://www.legalanalyticscourse.com/
https://landers.com.au/legal-insights-news/the-future-of-analytics-in-legal
https://www.knime.com/learning
https://github.com/Liquid-Legal-Institute/Legal-Text-Analytics
https://data.europa.eu/euodp/data/dataset/covid-19-documents-on-eur-lexTeaching methods
We provide slides, readings, exercises, technical manuals using https://virtuale.unibo.it/ and the TEAMS platform.
Considering the interdisciplinary approach and technical content, including the practice sessions, attendance is key.
This course requires an aptitude for pro-active participation with co-working skills.
Assessment methods
The exam requires you to design, implement, document (10 pages) a data-driven project using the competences acquired including the management of some software.
The project includes:
1. legal analysis;
2. ethics analysis;
3. technical analysis of AI methods and Data analytics models;
4. visualization of the results;
5. argumentation of the results.
The project could be carried out in groups of up to three people. The project must be presented orally with the possibility of discussing the theoretical part as well.
Teaching tools
We use the following support:
http://viruale.unibo.it for archiving material, slides, readings;
https://www.knime.com/ for developping scenarios;
https://www.r-project.org/ for statistical tools.
Office hours
See the website of Monica Palmirani
See the website of Salvatore Sapienza
SDGs
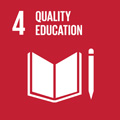
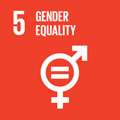
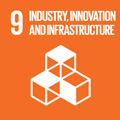
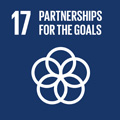
This teaching activity contributes to the achievement of the Sustainable Development Goals of the UN 2030 Agenda.