- Docente: Marilena Daquino
- Credits: 6
- SSD: M-STO/08
- Language: English
- Teaching Mode: Traditional lectures
- Campus: Bologna
- Corso: Second cycle degree programme (LM) in Digital Humanities and Digital Knowledge (cod. 9224)
-
from Nov 15, 2023 to Dec 14, 2023
Learning outcomes
At the end of the course the student knows principles and methods for knowledge acquisition, data sense making, and data visualization. The student will be able to manipulate existing datasets, especially Linked Open Datasets, and perform tasks such as: querying, filtering, normalising, transforming data into suitable data formats for data analysis purposes. Secondly the student will be able to select representative charts for answering research questions through visual graphics. Lastly, the student will be able to create web applications that leverage data storytelling techniques and show results of the data analysis.
Course contents
At the end of the course the student knows principles and methods of knowledge acquisition, data reengineering, sense making, and data visualization. The student is able to query and manipulate existing online datasets, organise them according to existing vocabularies, transform them into Linked Open Data, explore and analyse contents via quantitative methods, and create web applications that leverage data storytelling techniques.
The course is organised in lectures. Each lecture discusses one aspect of the pipeline for creating multi-purpose digital resources that leverage Linked Open Data, and includes references to existing tools and resources. A non-mandatory bibliography is provided during classes (references in the slide presentations) and as an annex to the course repository (see below, Readings/Bibliography).
Hand-on classes are provided in a tutorial-fashion, giving real-world examples of data manipulation, analysis, and web development, that students can use and extend for the sake of their project. Tutorials leverage well-known programming languages (e.g. Python, Javascript) frameworks, tools, and libraries, namely:
- jupyter notebook: for reproducibility and code documentation (alternatively, google colab notebooks can be used)
- python libraries for data manipulation and analysis: pandas, numpy, seaborn, mathplotlib
- python libraries for RDF data manipulation: RDFlib, SPARQLWrapper, requests
- javascript libraries for UI/UX and data visualization: JQuery, Google charts, D3.js
- github: for collaborative coding, storage, versioning, and web publication
Basic knowledge of Python, Github, RDF, and web languages (HTML, CSS, JS) is required. In detail, students should be able to confidently do the following:
- [STRONGLY RECOMMENDED] manipulate CSV and JSON data with Python libraries
- [RECOMMENDED] access and upload/update code on github
- [STRONGLY RECOMMENDED] create static web pages (from scratch or by means of templates)
- [RECOMMENDED] use Javascript to modify the DOM and/or to address User Interface problems
The lectures [L] and hands-on classes [H] are organised as follows:
- [L] Course introduction
- [L] Preliminary concepts on Data and Data visualization strategies
- [L/H] Preliminaries of Semantic Web
- [L/H] Data access and extraction
- [L/H] Data sense making and data exploration
- [H] Languages and libraries for data analysis: Jupyter notebook, Data exploration
- [L/H] Web development and Client-side libraries for data visualisation
- [L/H] Communication strategies and digital storytelling techniques
- [L/H] Seminar / to be defined
- [L] Publication, tools and best practices. Course recap, evaluation grid, Q&A
Readings/Bibliography
Lecture notes will be freely available from a dedicated GitHub repository before the course starts (please check this page before the beginning of the course for further information).
Slides and any additional material will be made available a few days before each lecture in the same repository.
Suggested readings are provided during classes. References are also shared here and here.
Teaching methods
16-hour lectures, 14-hour hands-on classes.
Assessment methods
Students are required to present either a group web project or an individual web project leveraging data and technologies shown during the course (or other compatible technologies despite not discussed in depth during the course). Every year general topics are proposed by the teacher. Students have to develop their own research questions (based on the proposed topic) and produce data-driven analysis and stories. This year topics include: art history, history of photography, gender studies. Specifications of the project are detailed in the lecture notes (see GitHub repository).
The exam consists of a 15-minute presentation of the project, followed by question and answering.
In case of group projects, single students’ contributions to the project will be evaluated.
The evaluation grid will be discussed during the course and is published on the course GitHub repository.
The same program applies to not attending students.
Teaching tools
Students must be able to access course classes that are available on GitHub (https://github.com/marilenadaquino/information_visualization). By the end of the course students should create a GitHub account (free of charge) in order to publish the web project.
Students must have installed an updated version of python (the latest if possible) on their personal computers and have chosen a preferred rich text editor. The teacher will show examples by using Atom editor. VS Code, PyCharm, SublimeText 2.0, or similar editors are also valid solutions.
Classes are recorded and streamed at this link Teams.
Recordings are available at this link.
Links to further information
https://github.com/marilenadaquino/information_visualization
Office hours
See the website of Marilena Daquino
SDGs
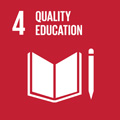
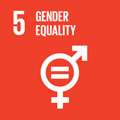
This teaching activity contributes to the achievement of the Sustainable Development Goals of the UN 2030 Agenda.