- Docente: Michaela De Giglio
- Credits: 6
- SSD: ICAR/06
- Language: Italian
- Teaching Mode: Traditional lectures
- Campus: Bologna
- Corso: Second cycle degree programme (LM) in Geography and Territorial Processes (cod. 0971)
-
from Sep 21, 2022 to Oct 28, 2022
Learning outcomes
At the end of the course students will acquire the following skills: digital image processing, the extraction of thematic information about several land and environmental aspects (geomorphology, urban, connected to cultural heritage, monitoring, etc..) and the management of remotely sensed data in remote sensing and GIS softwares.
Course contents
MODULE 1: BASIC PRINCIPLES OF REMOTE SENSING
- Remote Sensing introduction: definition, general system aspects, applications. Physical principles (from radiant energy to radiance, electromagnetic wave, wavelength, electromagnetic spectrum).
- Energy interactions with the atmosphere (reflection, transmission and emission). Atmospheric windows. Spectral signature: definition and description of water, soils and rocks, vegetation signatures.
- Observation tools: platforms, orbits, active and passive sensors, acquisition parameters (IFOV, Swath, satellite elevation angle, sun elevation angle), cross-track, cross-track systems, matrix systems. Acquisition Mode.
- Description of sensors, digital images, multispectral shooting, data display, geometric resolution, spectral resolution (pan-sharpening), radiometric resolution, time resolution.
MODULE 2: DISTURBANCES AND CORRECTION
- Radiometric and geometric image distortions. Geometric distortion sources (earth bending, rotation, altitude variation, speed, trim, panoramic distortion).
- Parametric and non-parametric geometric correction models (notes). Orthorectification (DTM), georeferencing. Main current missions.
MODULE 3: CLASSIFICATION
- Gaussian distribution.
- Classification theory. Pixel classification, supervised classification (preliminary steps, classification algorithms).
- Main supervised algorithms (minimum distance from the average, maximum likelihood, support vectore machine and others); Hard and soft algorithms (Bayes, Fuzzy notes).
- Unsupervised classification techniques (cluster, k-means, isodata). Pixel-oriented classification.
- Accuracy evaluation.
- Machine learning notes.
- PCA, image enhancement techniques, vegetation index.
Readings/Bibliography
Attending and non attending students
Lecture notes by the teacher.
Further reading:
Principi e metodi di telerilevamento. Pietro Alessandro Brivio, Giovanni Lechi, Eugenio Zilioli. Città studi edizioni.
Basics of Geomatics. Mario A. Gomarasca. Springer.
Teaching methods
In-presence lessons, supported by the use of slides, available among the didactic materials. At the beginning of the course, the teacher will provide all the information relating to the program, the texts and the bibliography and the necessary indications for the exam. However, in the last part of the course, practical exercises are foreseen through an open source software), during which attending students will be able to apply, under the teacher’s supervision, the data processing methodologies learned in the previous theoretical lessons.
Assessment methods
Attending and non attending students
Oral test. During the exam, the professor will assess the candidate's comprehension and cross knowledge of the topics covered by the program. Also, the student will be required to apply the remote sensing fundamental concepts to real cases and to make independent judgments. To access the exam, the student must create a map by applying one or more classification techniques on one digital satellite data (provided by the teacher or chosen by the candidate).
IMPORTANT: the presentation of the classification map only will be not sufficient to exceed the exam
The evaluation criteria will contribute to determining the final grade will be:
1) the level of knowledge, deepening and critical understanding of the contents;
2) the ability to argue, establish relationships between topics and apply theoretical principles to real cases;
3) the use of appropriate basic technical terminology;
4) the robustness of the reasoning that led to the creation of the classification map.
The final grade will be assigned according to the following evaluation scale:
- 18-21: sufficient performance;
- 22-24: satisfactory performance;
- 25-27: good enough performance;
- 28-29: good performance;
- 30: very good performance;
- 30 cum laude: excellent performance.
Teaching tools
Educational laboratory of Geomatics/Informatic with individual PC workstations, Open Source and trade software for digital image processing; Open Source GIS software; projector
Office hours
See the website of Michaela De Giglio
SDGs
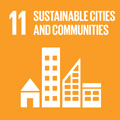
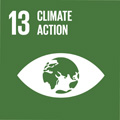
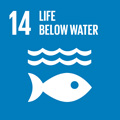
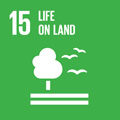
This teaching activity contributes to the achievement of the Sustainable Development Goals of the UN 2030 Agenda.