- Docente: Assimo Maris
- Credits: 6
- SSD: MAT/06
- Language: Italian
- Teaching Mode: Traditional lectures
- Campus: Ravenna
- Corso: Second cycle degree programme (LM) in Environmental Assessment and Management (cod. 8418)
Learning outcomes
Learning outcomes: knowledge of several topics in Applied Multivariate Statistical Analysis and capability to use the tools of Multivariate normal distribution for inference on population means, Multivariate analysis of Variance, Discriminant analysis, Multivariate regression, Cluster analysis, Prinicipal component analysis, and factor analysis.
Course contents
•Principles of R
• Data Organization. Sample multivariate descriptic statistics
• Random vectors and matrices. Mean vector and covariance matrix. Linear combinations of random vectors. Expected values and sample covariance matrix. Generalized and total sample variance
• Random Samples and the Expected Values of the Sample Mean and Covariance Matrix. Generalized Variance.
• Normal multivariate distribution: from the univariate to the multivariate case, fundamental properties and Contours of constant density. Multivariate Normal Likelihood. Large samples. Tests on normality: Q-Q plot. Transformation to normality.
• T2 di Hotelling: statistical hypotheses and level of significance, from the t-student to the T2 test. Confidence region and simultaneous confidence intervals for the mean vector of a normal distribution. Large samples. Charts for Monitoring a Sample of Individual Multivariate Observations for Stability.
• Paired comparisons. Comparing Mean Vectors from Two Populations. Comparing Several Multivariate Population Means: from ANOVA to MANOVA (with Wilks test).
• Classical multivariate regression model. Least squares estimation. Confidence intervals for the estimates. Model evaluation. Regression for new predictive variables.
• Principal component analysis (PCA). Analysis of data variability. Data reduction and compression of images.
• Similarity and distance measures. Cluster analysis with aggregation methods.
• Introduction to the machine learning systems: neural networks, evolutionary algorithms.
Readings/Bibliography
Applied Multivariate Statistical Analysis, R. A. Johnson e D. W. Wichern, Prentice Hall, V edizione, 2002
Introduzione alla chemiometria, Tedeschi Roberto, Edises, 1998
Metodi Statistici per la Sperimentazione Biologica, A. Camussi, F. Möller, E. Ottaviano, M. Sari Gorla, Zanichelli, II edizione, 1995.
Teaching methods
Classroom lectures and practical computer sessions.
As concerns the teaching methods of this course unit, all students must attend Module 1, 2 on Health and Safety online:
https://elearning-sicurezza.unibo.it/?lang=en
Assessment methods
Oral examination plus computer-practical exercise.
Teaching tools
Blackboard (lectures and exercises) and video-projector.
Computational laboratory practicals
Lecture notes.
Office hours
See the website of Assimo Maris
SDGs
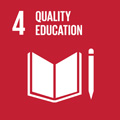
This teaching activity contributes to the achievement of the Sustainable Development Goals of the UN 2030 Agenda.