- Docente: Stefano Diciotti
- Credits: 6
- SSD: ING-INF/06
- Language: English
- Moduli: Stefano Diciotti (Modulo 1) Stefano Diciotti (Modulo 2)
- Teaching Mode: Traditional lectures (Modulo 1) Traditional lectures (Modulo 2)
- Campus: Bologna
- Corso: Second cycle degree programme (LM) in Electronic Engineering (cod. 0934)
-
from Mar 21, 2025 to Apr 29, 2025
-
from May 06, 2025 to Jun 13, 2025
Learning outcomes
At the end of the course, the student has: -knowledge regarding the need for AI in Medicine; -understanding of weaknesses of AI techniques, methods to overcome the challenges of AI in Medicine, and ways in which AI may improve healthcare; -essential knowledge to develop and implement reliable AI solutions in clinical research (using, e.g., supervised and unsupervised machine and deep learning techniques, explainable AI methods, and generative adversarial techniques); -practical skills to develop state-of-the-art AI tools with real-world medical data (e.g., clinical and imaging data); -the ability to critically read, discuss and evaluate methods and results of studies using AI in Medicine; -an overview of state-of-the-art AI-powered tools in Medicine.
Course contents
· Introduction to AI in Health and Medicine: progress, challenges, and opportunities;
· Introduction to Clinical Data: medical data collection and management, ethics and informed consent, quality control, data harmonization, data augmentation, synthetic datasets, dataset size vs. confidence intervals;
· Fundamentals of AI for Medicine: weaknesses of AI techniques and their consequences in Medicine, evaluation of AI tools in Medicine, explainable AI, AI reproducibility, beyond supervised learning, federated and swarm learning, transparent reporting, the ethics of AI in healthcare and Medicine, clinical integration;
· Hands-on Projects with Clinical Data: projects to provide technical experience in AI for Medicine;
· Real-world AI-powered tools of AI in Medicine: an overview of state-of-the-art AI solutions in Medicine and Healthcare.
Readings/Bibliography
Notes provided by the Professor.
M. Lutz, "Learning Python", O'Reilly, 2013
I. Goodfellow, Y. Bengio, A. Courville, "Deep Learning", MIT Press, 2016 (https://www.deeplearningbook.org/ )
Teaching methods
The course includes both ex-cathedra lessons and practical exercises carried out using Google Colab. The aim of the lectures is to provide the students with a solid theoretical knowledge in artificial intelligence for Medicine, and to raise awareness about the advantages and limitations of each available technique. The practical exercises are designed to train students in solving real biomedical problems, and to highlight the potential benefits and challenges introduced by artificial intelligence techniques and software tools.
Given the type of activity and teaching methods adopted, the attendance of this training activity requires the prior participation of all students in modules 1 and 2 of training on safety in the workplace [https://elearning-sicurezza.unibo.it/] in e-learning mode.
Assessment methods
The learning assessment will be performed through a final examination consisting of an oral test focused on both the theoretical concepts presented during the lessons and the software tools used in the laboratory. The exam aims to ascertain the student’s theoretical-practical skills and competences, the correctness of language, and the clarity of concepts and exposition.
As part of the final assessment, students are required to develop and present a software project using Python in Google Colab, applying artificial intelligence to solve a real-world biological or medical problem. The project may be selected from a list provided during the course or proposed independently by the student, subject to instructor approval. Projects may be completed individually or in pairs; in the latter case, the scope and complexity of the project must be appropriately increased.
The code must be implemented in Python and must run in Google Colab. It must be submitted as a .ipynb notebook. Students may submit their notebook in one of the following ways:
-
via a personal GitHub repository (provide the link),
-
via a Google Colab shareable link, or
-
by sending the file directly via email to the instructor.
In addition to the code, students must submit a project report in PDF format, prepared according to the official template available in the course’s GitHub repository or on the Virtuale platform.
Both the code and the report must be submitted at least 7 days before the scheduled exam date.
Teaching tools
Course GitHub repository (access available upon request to the instructor) containing project templates, notebooks, and other resources.
Use of Google Colab for coding and project development.
Materials provided by the instructor on the Virtuale platform, including lecture slides and selected scientific articles.
Office hours
See the website of Stefano Diciotti
SDGs
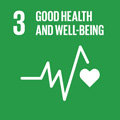
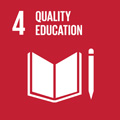
This teaching activity contributes to the achievement of the Sustainable Development Goals of the UN 2030 Agenda.