- Docente: Maria Elena Bontempi
- Credits: 6
- SSD: SECS-P/05
- Language: Italian
- Moduli: Maria Elena Bontempi (Modulo 1) Maria Elena Bontempi (Modulo 2)
- Teaching Mode: Traditional lectures (Modulo 1) Traditional lectures (Modulo 2)
- Campus: Forli
-
Corso:
First cycle degree programme (L) in
Economics and business (cod. 9202)
Also valid for First cycle degree programme (L) in Management and Economics (cod. 5892)
-
from Feb 11, 2025 to Mar 12, 2025
-
from Apr 09, 2025 to Apr 30, 2025
Learning outcomes
The aim of the course is to provide students with adequate knowledge of the basic econometric tools for empirical investigations of cross-sectional and time series data. Drawing on critical discussion about microeconomic and financial applications, students develop the basic skills to perform empirical work using econometric software. At the end of the course students are able to: (a) choose between different econometric models and estimation techniques; (b) discuss the empirical results of the economic and financial analyses proposed in class; (c) to perform one’s own analysis using econometric/statistical software.
Course contents
Learning objectives: at the end of this course, students will be able to: 1. Understand the role and importance of econometrics in economic analysis. 2. Formulate and estimate linear regression models. 3. Performe hypothesis testing and interpret statistical significance. 4. Identify and correct common econometric problems such as multicollinearity, heteroskedasticity, and endogeneity. 5. Use econometric software (Stata) for empirical analysis.
Course outline:
- What is econometrics? The research question, definition and scope of econometrics. The different types of data: cross-sections, time-series, panel data.
- Understanding data through exploratory data analysis: distributions, tables, histograms, scatterplots, hypothesis testing and joint hypothesis testing.
- The classical linear regression model (CLRM) and the OLS estimator: assumptions and properties of the method.
- Application of the OLS to the simple regression model: validation of the method through specification tests on residuals; interpretation of the coefficients derived from the estimation, confidence intervals, goodness of fit.
- Modification of the functional form (logarithms and quadratic forms).
- Dummy variables and interaction terms: categorical variables in regression; interaction effects and interpretation.
- Extension to multiple regression models: interpretation of coefficients; omitted variables bias; multicollinearity and its consequences.
- What to do when OLS assumptions are no longer valid? Heteroskedasticity is an example of OLS assumption violation: detection and remedies. Robust standard errors. Generalised least squares.
- Another violation of OLS assumptions: endogeneity of explanatory variables. Sources of endogeneity. The instrumental variables approach.
Clearly there are necessary prerequisities, specifically for Erasmus students:
1. At this page [https://corsi.unibo.it/1cycle/Management/course-structure-diagram/piano/2024/5892/000/000/2024] take a look at the content of Statistics and Business Statistics
2. A knowledge, at least basic, of STATA software is also required
Readings/Bibliography
The material (articles, commented notes & slides, programs and data-sets) will be distributed during the lectures and make available on the platform Virtuale.
The reference textbook is:
Wooldridge J.M. 2020 Introductory Econometrics. A Modern Approach, Cengage, 7th Edition.
Why programming in Stata? Have a look at Cox N. J. (2001) Speaking Stata: How to repeat yourself without going mad, The Stata Journal, 1, Number 1, pp. 86–97
Teaching methods
To ensure a smooth transition from theory to practice in econometrics, theoretical lectures are combined with working sessions. During the practical empirical applications, you will use the computer and Stata econometric software (available with a CAMPUS licence and your university credentials).
At the end of the course, you will be able to critically evaluate articles that present basic empirical analyses and to model and estimate your own regression of interest, using the most appropriate methods according to the problem you face.
Assessment methods
Attending students: some homework will be assigned during the course. These ‘exercises’ are intended to reinforce the concepts covered in class, to replicate, using new data, the empirical analyses/guided exercises carried out together in class, to familiarise you with the software (which is a professional tool) and, above all, to understand how to interpret the results, i.e. how to prepare for the individual exam. Students can work alone or in groups of up to 4 participants. Overall, homework accounts for 40% of the final grade.
The remaining 60% of the grade will be individual, through a final exam to be taken in class, after registering on AlmaEsami. The final exam will take place on the EOL platform, where you will find a STATA output with questions about the results, which you will have to answer with your comments and considerations. Your ability to interpret will obviously be strengthened by having done the homework.
Non-attending students will be assessed only on the individual exam and must be prepared on the entire course programme, which can be consulted on Virtuale.
The final grade may be:
30L excellent work!
28-30: independent knowledge and skills leading to good understanding and analytical ability.
24-27: the degree of independent knowledge is appreciable
18-23: rather random answers, lacking structure or logical order, with theoretical and methodological inaccuracies.
<18: incorrect, copied or missing answers
Teaching tools
Theoretical lectures are associated with working sessions; during them you will receive the suggestions needed to run your own empirical analysis. The data-sets and the programming files to perfom applied analyses will be provided during the lectures. The distributed material will be make available on the Virtuale platform. A virtual room on TEAMS will be available in case you cannot physically attend a lecture and to communicate via chat.
Office hours
See the website of Maria Elena Bontempi
SDGs
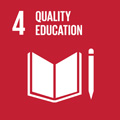
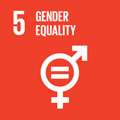
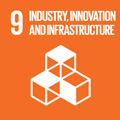
This teaching activity contributes to the achievement of the Sustainable Development Goals of the UN 2030 Agenda.