- Docente: Gastone Castellani
- Credits: 6
- SSD: FIS/07
- Language: English
- Moduli: Gastone Castellani (Modulo 1) Daniel Remondini (Modulo 2) Nico Curti (Modulo 3)
- Teaching Mode: Traditional lectures (Modulo 1) Traditional lectures (Modulo 2) Traditional lectures (Modulo 3)
- Campus: Bologna
-
Corso:
Second cycle degree programme (LM) in
Science of Climate (cod. 5895)
Also valid for Second cycle degree programme (LM) in Physics (cod. 9245)
-
from Feb 29, 2024 to Apr 04, 2024
-
from Apr 05, 2024 to May 10, 2024
-
from May 17, 2024 to May 28, 2024
Learning outcomes
The student will learn the basis of artificial intelligence and machine learning (clustering, classification, regression, neural networks and Bayesian methods) and its application to climate science, with particular attention to the analysis of time series and space-resolved data. The student will learn how to implement these methods in open-source environments (eg. Python and R).
Course contents
Introduction Probability Theory, Probability densities, Expectations and covariances, Bayesian probabilities, Bayesian curve fitting, Model Selection, the Curse of Dimensionality, Statistical Inference and decision, loss functions for regression
Probability Distributions Binary and Multinomial Variables, Beta, Dirichlet and Gaussian Distribution, Gaussian Mixtures, the Exponential Family, maximum likelihood and sufficient statistics, Conjugate priors, Noninformative priors, Nonparametric Methods Inference and association test
Linear models for Regression and Classification Linear Basis Function Models, Bias-Variance Decomposition, Bayesian Linear Regression, Bayesian Model Comparison, Discriminant Functions, Probabilistic Generative and Discriminative Models
Neural Networks Learning rules, Hebbian,BCM and Hopfield model, Feed-forward Network Functions, Network Training, Error Backpropagation, Regularization,
Introduction to Kernel methods and Graphical models
Feature extraction & optimal projection:
Principal Component Analysis, Singular Value Decomposition, Factor Analysis, Multi-Dimensional Scaling, ISOMAP, Relation to cost function maximization and eigenvalue problem (Rayleigh quotient), Practical aspects: data regularization and normalization.
Supervised classification:
Support Vector Machine, Discriminant Analysis, Random Forest.
Unsupervised clustering:
Hierarchical, k-means, spectral clustering techniques.
Introduction to Deep Learning:
Error minimization: the backpropagation algorithm.,Feedforward Neural Networks., Convolutional Neural Networks.
Robust analysis
Bias-variance dilemma, Best practices, Crossvalidation procedures
Suggested books:
Readings/Bibliography
Bishop - Machine learning and pattern recognition
Tibshirani Tusher - Methods of statistical learning
Teaching methods
Slides and blackboard
Assessment methods
Project, literature study and questions.
Teaching tools
Programming environment and server connection.
Office hours
See the website of Gastone Castellani
See the website of Daniel Remondini
See the website of Nico Curti
SDGs
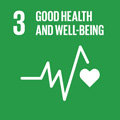
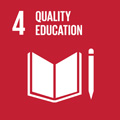
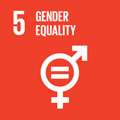
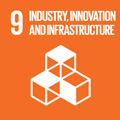
This teaching activity contributes to the achievement of the Sustainable Development Goals of the UN 2030 Agenda.