- Docente: Enrico Gallinucci
- Credits: 6
- SSD: ING-INF/05
- Language: English
- Moduli: Enrico Gallinucci (Modulo 1) Enrico Gallinucci (Modulo 2)
- Teaching Mode: Traditional lectures (Modulo 1) Traditional lectures (Modulo 2)
- Campus: Cesena
-
Corso:
Second cycle degree programme (LM) in
Computer Science and Engineering (cod. 8614)
Also valid for Second cycle degree programme (LM) in Digital Transformation Management (cod. 5815)
-
from Sep 19, 2023 to Oct 25, 2023
-
from Sep 20, 2023 to Dec 12, 2023
Learning outcomes
At the end of the course, the student:- Knows the applications of Big Data technologies and the respective challenges - Knows the available hardware and software architectures to handle Big Data - Knows the techniques to store the data, the programming languages and paradigms generally adopted in this kind of systems - Knows the design methodologies for the different kinds of applications in the area of Big Data - Acquires practical expertise in using the different technologies through laboratory and projects. In particular, the main technologies used in practical exercises will be NoSQL databases and the Hadoop platform: Hive, Spark, Tez, Dremel, Giraph, Storm, Mahout, and Open R
Course contents
Requirements
A prior knowledge of relational databases, Java and Scala programming languages, and Unix-like systems is required to attend the course. Attendance of Business Intelligence and Data Mining courses is encouraged.
Classes and teaching material are in English. A good comprehension of English is thus required to use the material and interact during class. Exam can be given in Italian.
Modules
The course is split into two modules.
The first module (20 hours) is mostly theoretical and it is shared with the Master's Degree in Digital Transformation Management (corresponding to Module 1 of Big Data and Cloud Platforms [https://www.unibo.it/it/didattica/insegnamenti/insegnamento/2022/466768] ).
The second module (30 hours) is mostly practical and it is exclusive to the Master's Degree in Computer Science and Engineering.
Course Contents
1. Introduction to the course and to Big Data: what they are and how to use them
2. Cluster computing to handle Big Data
- Hardware and software architectures
- The Apache Hadoop framework and its modules (HDFS, YARN)
- Hadoop-specific data structures (Apache Parquet)
3. The MapReduce paradigm: basic principles, limitations, design of algorithms
4. The Apache Spark system
- Architecture, data structures,basic principles
- Data partitioning and shuffling
- Optimization of the computation
5. SQL on Big Data with Spark SQL
6. Data streaming
- The architecture to handle data streaming
- Approximated algorithms in the streaming context
7. NoSQL databases
8. Handling Big Data in the Cloud
- Cluster on-premises vs in the cloud
- The technological stack in the cloud
- Deploy of a real case study on a cloud provider
9. Designing non-trivial problems under the MapReduce paradigm
Readings/Bibliography
- Slides
Recommended readings:
- Tom White. Hadoop - The Definitive Guide (4th edition). O'Reilly, 2015
- Matei Zaharia, Holden Karau, Andy Konwinski, Patrick Wendell. Learning Spark, 2nd Edition. O'Reilly, 2020
- Andrew G. Psaltis. Streaming Data - Understanding the real-time pipeline.Manning, 2017
- Ian Foster, Dennis Gannon. Cloud Computing for Science and Engineering. MIT Press, 2017
Further readings will be mentioned during the course.
Teaching methods
Lessons and practical exercises.
As concerns the teaching methods of this course unit, all students must attend Module 1, 2 on Health and Safety [https://www.unibo.it/en/services-and-opportunities/health-and-assistance/health-and-safety/online-course-on-health-and-safety-in-study-and-internship-areas] online.
Assessment methods
The exam consists in an oral examination on all the covered topics and in the discussion of a project.
Project details:
- Goal: identifying a big-enough dataset and designing a notebook application to process and analyze the data (using the techniques and tools learned throughout the course). Both the dataset and the workload must be validated by the teacher before beginning the implementation.
- Groups of up to 2 people can be formed.
- The discussion is done on the day of the oral examination.
- A well-done project can improve the vote obtained with the oral examination; a bad one can impede the access to the oral examination.
- Alternative proposals for projects (e.g., in combination with projects of other courses or with the internship/thesis) are always well-received.
Teaching tools
Practical exercises will be carried out on the cloud-based virtual environment provided by the AWS Academy service. Students registering to AWS Academy will receive 100$ to spend on AWS services, which will be enough to carry out both the exercises during class and the project. SSH tunnelling will be necessary to connect to the GUI of the cloud services (e.g. Spark).
Office hours
See the website of Enrico Gallinucci
SDGs
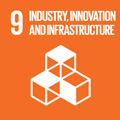
This teaching activity contributes to the achievement of the Sustainable Development Goals of the UN 2030 Agenda.