- Docente: Mauro Gaspari
- Credits: 6
- Language: English
- Teaching Mode: Traditional lectures
- Campus: Bologna
- Corso: Second cycle degree programme (LM) in Quantitative Finance (cod. 8854)
Learning outcomes
The student is exposed to selected frontier issues of research from scholars in each field. Each scholar will address a topic, starting from the basic principles to the frontier questions. From the workshop, the student will collect ideas for his thesis and interests driving him to his future career.
Course contents
The program of the QF workshop this year is focused on technological aspects dealing with advanced time series analysis exploiting artificial intelligence, natural language processing for financial applications, cryptocurrencies and security.
The final schedule will be published in March 2023. The workshop will be held in the last week of May.
The following seminars will be held:
Title: The Dark Side of Digital Revolution (4 hrs)
Prof. Nicola Dragoni, DTU Denmark.
Teaser: Click here to kill everybody: the dark side of digital revolution
Abstract: The rise of connectivity and digitalization, combined with the race for bringing artificial intelligence into every aspect of our daily life, is rapidly changing our society and shaping its future development. In particular, the Internet of Things (IoT) revolution is creating a world in which everyday objects are connected to the Internet, making computing pervasive like never before. In this technological and societal revolution, cybersecurity has dramatically been too neglected, exposing society to a potential safety (life-threatening) and security disaster. This seminar will give a high level security perspective on the ongoing digital revolution, showing how hackers can control our life if we don’t urgently take cybersecurity seriously into consideration.
Title: Demystifying and Demythologizing Blockchain Technology (4 hrs)
Prof. Nicola Dragoni, DTU Denmark.
Teaser: Blockchain: disruptive technology or one-hit wonder?
Abstract: This seminar will give a gentle, conceptual and honest introduction to blockchain technology. Gentle means that the seminar is targeted to non experts. Conceptual means the focus will be on key concepts instead of technicalities or one specific blockchain platform. Last but not least, honest means that the seminar will not be the (usual) enthusiastic business talk about blockchain, but it will highlight misconceptions and dispel some common myths about blockchain. Examples will be based on cryptocurrency technologies and decentralised finance.
Title: Linear and non-linear stochastic models: theory and application to financial market (2 hrs). Wavelet theory and financial time series (2 hrs)
Prof. Livio Fenga, University of Exeter (UK)
Abstract: Non-linear but also linear models can be effectively used in quantitative finance. Under certain conditions (e.g., low frequency and low roughness’ levels) even linear models can adequately perform on financial time series. In this session those models will be illustrated and applied using the software R.
Stock market’s noisy, non-stationary and non-linear characteristics can be dealt with by framing the analysis in Scale - Time domain (Wavelets). This is a useful approach in many circumstances, e.g., for the extraction of the relevant information and for denoising purposes. The Heisenberg's quantum principle of uncertainty is also presented and discussed.
Title: Self-similarity, fractals, and chaos in financial time series (2 hrs). AI models for the prediction of stock market data (2 hrs)
Prof. Livio Fenga, University of Exeter (UK)
Abstract: In this session the fundamentals of important concepts, such as chaos theory, quantum mechanic and long memory are introduced. The inconsistencies and failures of the Neoclassical Theory in financial markets will be also highlighted in the light of new quantum paradigms.
Title: Multivariate analysis of stock market data using big data regressor matrices (2 hrs)
Prof. Livio Fenga, University of Exeter (UK)
Abstract: Financial data are often investigated using secondary information, such as sentiment data (e.g., Twitter) and, in general, big data. However, the use of additional data sources can increase the set of the explanatory variables to a point that the extraction of the relevant information can become a daunting task. In this session, multivariate techniques and reduction methods will be presented.
Title: Machine learning in forecasting energy prices and volatility
Prof. Nikola Gradojevic, University of Guelph, CANADA
Abstract: An accurate forecasting model for energy commodities (e.g., natural gas, crude oil, and electricity) pricing and forecasting is essential not only for trading, but also for commodities’ production, storage, and distribution. Economic planning, investments in the energy sector, and environmental protection represent additional activities that may rely on accurate price and volatility forecasting models. Such models could also assist government policy-makers in their planning efforts for electric power production and market design.
This seminar will present non-linear, machine learning model architectures that allow for rather complex relationships between predictors and the dependent variable (energy commodities’ returns and volatility), non-normality of price return distributions and, also, facilitate adaptive learning. To explain the complex non-linear relationships inherent in price and volatility forecasting, the explainable artificial intelligence (XAI) method will be used.
Title: Introduction to Natural Language Processing for financial applications (4 hrs)
Prof. Pierpaolo Basile, University of Bari
Abstract: Natural language processing (NLP) is a subfield of computer science - specifically, the branch of artificial intelligence - which aims to give computers the ability to understand written and spoken words in the same way human beings can.
The seminar introduces the basic concepts and tasks involving NLP methods.
Title: Introduction to Information Extraction for financial applications (4 hrs)
Dott. Marco Polignano, University of Bari
Abstract: Information extraction (IE) systems automatically extract structured information from unstructured or semi-structured machine-readable sources. Generally, this activity concerns processing human language texts through natural language processing (NLP) techniques. The output of the extraction processing is a set of structured information stored in databases, ontologies, or knowledge graphs.
The seminar aims to provide an overview of the information extraction problem and state-of-the-art approaches to dealing with it. Moreover, some real-case scenarios in which IE approaches are exploited will be proposed.
Readings/Bibliography
3) Linear and non-linear stochastic models, Wavelet theory
Graves, Spencer. 2014. FinTS: Companion to Tsay (2005) Analysis of Financial Time Series. https://CRAN.R-project.org/package=FinTS [https://cran.r-project.org/package=FinTS] .
Donald B.P., Andrew T.W., Wavelet methods for time series analysis. Cambridge University Press, 2000, p 150.
4) Self-similarity, fractals, and chaos, AI models for the prediction
Bianchi, Sergio and Pianese, Augusto. (2007). Modeling stock price movements: Multifractality or Multifractionality? Quantitative Finance, vol.7, Nº. 3 (June) pp. 301-319.
Blackledge, Jonathan M. (2008). Application of the Fractal Market Hypothesis for Macroeconomics Time Series Analysis. isast Transactions on Electronic and Signal Processing. Nº. 1 vol. 2. Blackledge J.M., p. 89
5) Multivariate Analysis
Hastie, Trevor, Robert Tibshirani, and Martin Wainwright. "Statistical learning with sparsity." Monographs on statistics and applied probability 143 (2015): 143.
Roy, S.S., Mittal, D., Basu, A., Abraham, A. (2015). Stock Market Forecasting Using LASSO Linear Regression Model. In: Abraham, A., Krömer, P., Snasel, V. (eds) Afro-European Conference for Industrial Advancement. Advances in Intelligent Systems and Computing, vol 334. Springer, Cham. https://doi.org/10.1007/978-3-319-13572-4_31
6) Machine learning in forecasting energy prices and volatility
Čeperić, E., Žiković, S., and Čeperić, V. (2017). Short-term forecasting of natural gas prices using machine learning and feature selection algorithms. Energy, 140, 893–900.
Bajatović, D., Erdemlioglu, D., and Gradojevic, N. (2023). Drilling deeper: Non-linear, non-parametric natural gas price and volatility forecasting, forthcoming in The Energy Journal.
Berrisch, J. and Ziel, F. (2022). Distributional modeling and forecasting of natural gas prices. Journal of Forecasting, 41(6), 1065–1086.
Alexandre Bonnet R. Costa et al. (2021). Machine learning and oil price point and density forecasting, Energy Economics, 102, 105494.
Gradojevic, N., Kukolj, D., Adcock, R., and Djakovic, V. (2023). Forecasting Bitcoin with technical analysis: A not-so-random forest?, forthcoming in International Journal of Forecasting.
Uribe, J. M., Guillen, M., and Mosquera-López, S. (2018). Uncovering the nonlinear predictive causality between natural gas and electricity prices. Energy Economics, 74, 904–916.
Wagner, A. Ramentol, E., Schirra, F., and Michaeli, H. (2022). Short- and long-term forecasting of electricity prices using embedding of calendar information in neural networks, Journal of Commodity Markets, 100246.
7) Natural Language Processing
Dan Jurafsky and James H. Martin. Speech and Language Processing (3rd ed. draft). Online: https://web.stanford.edu/~jurafsky/slp3/.
Gao, Ruizhuo, et al. "A review of natural language processing for financial technology." International Symposium on Artificial Intelligence and Robotics 2021. Vol. 11884. SPIE, 2021.
Xing, Frank Z., Erik Cambria, and Roy E. Welsch. "Natural language based financial forecasting: a survey." Artificial Intelligence Review 50.1 (2018): 49-73.
Fisher, Ingrid E., Margaret R. Garnsey, and Mark E. Hughes. "Natural language processing in accounting, auditing and finance: A synthesis of the literature with a roadmap for future research." Intelligent Systems in Accounting, Finance and Management 23.3 (2016): 157-214.
8) Information Extraction
Jacobs, Gilles, and Véronique Hoste. "SENTiVENT: enabling supervised information extraction of company-specific events in economic and financial news." Language Resources and Evaluation 56.1 (2022): 225-257.
Costantino, Marco, and Paolo Coletti. Information extraction in finance. Vol. 8. Wit Press, 2008.
Saggion, Horacio, et al. "Ontology-based information extraction for business intelligence." The Semantic Web. Springer, Berlin, Heidelberg, 2007. 843-856.
Costantino, Marco, et al. "Natural language processing and information extraction: Qualitative analysis of financial news articles." Proceedings of the IEEE/IAFE 1997 Computational Intelligence for Financial Engineering (CIFEr). IEEE, 1997.
Teaching methods
Seminars. Attendance is mandatory, at least 75% of all classes is necessary and will be verified.
Assessment methods
Discussion of a scientific paper.
The exam will be evaluated on a score ranging up to 30.
Teaching tools
Slides and blackboard
Office hours
See the website of Mauro Gaspari
SDGs
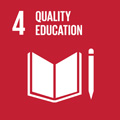
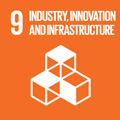
This teaching activity contributes to the achievement of the Sustainable Development Goals of the UN 2030 Agenda.