- Docente: Elisa Magosso
- Credits: 6
- SSD: ING-INF/06
- Language: English
- Teaching Mode: Traditional lectures
- Campus: Cesena
-
Corso:
Second cycle degree programme (LM) in
Biomedical Engineering (cod. 9266)
Also valid for Second cycle degree programme (LM) in Biomedical Engineering (cod. 9266)
Learning outcomes
At the end of the module, the student knows methods for scalp EEG signal processing, to analyze the ongoing EEG activity and its changes related to specific sensory/motor/cognitive events. In particular, the student knows techniques for: i) artefact removal from the scalp EEG signals, ii) extraction of Event-Related-Potentials (ERP), iii) time-frequency representation, iv) estimation of cortical activations from the scalp EEG potentials (solution of the problem inverse), v) estimation of connectivity between brain regions. The student will be able to use software packages that implement these techniques in a correct and critical way and will be able to properly interpret the obtained results.
Course contents
Physiological Basis of the EEG signal
- Neural potentials and origin of the scalp EEG signal
- Brain rhythms and Event-Related Potentials (ERP)
- Main artifacts in the EEG signal
Preprocessing of the EEG signal
- Filtering, resampling, re-referencing
- Extraction of epochs in trial-based EEG recordings
- Artifact removal via Independent Component Analysis
Analysis in the time, frequency and time-frequency domains
- The Event-Related Potentials (ERPs): averaging technique and components of the ERP
- Power spectral density and power in specific frequency bands
- Time-frequency analysis via Wavelet Transform
Reconstruction of cortical sources
- The forward problem (from cortical activations to scalp EEG potentials) and head models
- The inverse problem (from scalp EEG potentials to cortical activations): single-dipole (or a few-dipole) models and source distributed models
- Approaches to solve the invers problem
Brain connectivity analysis
- Introduction to brain connectivity and definitions of connectivity
- Methods for estimating brain connectivity, with particular reference to 'Granger Causality'
Artificial intelligence approaches applied to decoding the EEG signal
- Decoding of EEG signal: problem definition
- Approach to solve the EEG decoding problem via deep learning based on convolutional neural networks and interpretation of results
Introduction to statistical analysis
Laboratory Activities
- Acquisition of EEG signal by the electroencephalographic system available in the Biomedical Engineering Lab (LIB)
- Use of Matlab and specific toolboxes (e.g. EEGLAB) in computer lab, to analyze EEG data by applying the theoretical concepts exposed
Readings/Bibliography
- Lecture notes of the teacher available on “IOL-Insegnamenti On Line”
- R. Hari and A. Puce, “MEG-EEG Primer”, Oxford University Press
- M.X. Cohen, “Analyzing neural time series data: Theory and Practice”, MIT Press
- R.J. Ilmoniemi and J. Sarvas, “Brain Signals: Physics and Mathematics of MEG and EEG”, MIT Press
Teaching methods
The course is organized into in-class lectures and laboratory activities. The lectures aim to provide the theoretical knowledge to understand and possibly implement the techniques for EEG signal analysis. Lab activities (mainly carried out in computer lab) allow the student to learn the software tools (in particular Matlab toolbox) to apply the presented techniques, studying the obtained results, improving the understanding of these techniques and developing critical sense in their use.
As concerns the teaching methods of this course unit, all students must attend Module 1, 2 [https://www.unibo.it/en/services-and-opportunities/health-and-assistance/health-and-safety/online-course-on-health-and-safety-in-study-and-internship-areas] on Health and Safety online.
Assessment methods
Student learning assessment will be performed through a final examination consisting of a oral test. The oral test focuses both on the theoretical concepts presented during the lessons and on the software tools used in lab, and ascertains the theoretical-practical skills and competences of the student, correctness of language, clearness of concepts and exposition.
Examination dates will be fixed on the basis of School calendar and will be published on Almaesami (https://almaesami.unibo.it/almaesami/welcome.htm ). Students can register for the examination only through the Almaesami service (https://almaesami.unibo.it/almaesami/welcome.htm )..
Teaching tools
Blackboard, document camera, notebook, projector, lecture notes, Biomedical Engineering Laboratory, Computer Laboratory, Matlab and specific toolboxes
Office hours
See the website of Elisa Magosso
SDGs
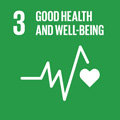
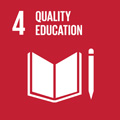
This teaching activity contributes to the achievement of the Sustainable Development Goals of the UN 2030 Agenda.