- Docente: Michele Scagliarini
- Credits: 8
- SSD: SECS-S/01
- Language: Italian
- Moduli: Michele Scagliarini (Modulo 1) Gabriele Soffritti (Modulo 2)
- Teaching Mode: Traditional lectures (Modulo 1) Traditional lectures (Modulo 2)
- Campus: Bologna
- Corso: First cycle degree programme (L) in Statistical Sciences (cod. 8873)
Learning outcomes
By the end of the course the student gains an appreciation of the types of problems and questions which arise with multivariate data and the basic theory of survey sampling.
In particular the student should be able:- to apply and interpret methods of dimension reduction (including principal component analysis and factor analysis); - to apply and interpret methods for cluster analysis and discrimination; - to interpret the output of R procedures for multivariate statistics - to employ simple, stratified and probability sampling; - to derive the estimators and associated standard errors of population in the different sampling strategies; - to correct estimation by the ratio principle; - to understand the difference between observational and experimental studies.
Course contents
Module 1: Survey Sampling
Michele Scagliarini
1. Introduction, Population and Sample (4 hours).
-General introduction
-Sampling from finite populations
-Definition of population and descriptive statistics
-Definition of sample and descriptive statistics
2. Random sampling and estimators (4 hours).
-Definition of sampling design: extraction and inclusion probabilities
-Linear homogeneous estimators
-exercises
3. Simple random sampling (4 hours).
-simple random sampling with replacement
-simple random sampling without replacement
-exercises
4. Sampling with varying probability (6 hours).
-the Horvitz Thompson estimator
-the Hansen Hurwitz estimator
-exercises
5. The use of auxiliary information variables in simple random sampling (4 hours).
-the ratio estimator
-the regression estimator
-exercises
6. Stratified random sampling (5 hours)
-Optimal allocation
-Proportional allocation
-exercises
7. Final exercises (3 hours)
Module 2: Data Analysis
Gabriele Soffritti
-
Data matrices and additional matrices useful for multivariate statistical analysis (6 hours)
-
Cluster analysis (6 hours)
Hierarchical Clustering
K-means Clustering -
Principal component analysis (6 hours)
Geometrical concepts and mathematical details
Properties and practical considerations -
Factor analysis (6 hours)
The linear factor model: specification, identification and estimation
Rotation methods
Factor scores -
Discriminant analysis (6 hours)
Discrimination when the populations are known (maximum likelihood and Bayes discriminant rules)
Fisher's linear discriminant function
Estimation of the error rate -
Functions for performing principal component analysis, factor analysis, cluster analysis, discriminant analysis in the R environment (8 hours)
Syntax, functionalities and output
Illustrative examples carried out in R with comments on the obtained results
The estimated number of hours of lessons for each topic takes account of the additional practical lessons that will take place in a computer laboratory on a weekly basis starting from the second week (November 18-22, 2019; teacher: Dott. Gabriele Perrone).
Readings/Bibliography
Module 1: Survey Sampling
Downloadable lecture notes: Daniela Cocchi "Teoria dei Campioni (corso base)"
Sharon Lohr, “Sampling: design and analysis”, Pacific Grove, Duxbury press, 1999.
Some additional readings will be indicated during the course.
Additional useful readings
Module 2: Data Analysis
Compulsory readings
-
S. Mignani, A. Montanari, Appunti di analisi statistica multivariata. Esculapio, Bologna, 1994. Chapters 3, 4, 5, 7.
-
Teacher's lecture notes with examples of exercises from past written exams.
-
Additional teaching material will be provided by the teacher during the lessons.
Teacher's lecture notes are available on the platform "Insegnamenti online - Supporto online alla didattica" (IOL) (https://iol.unibo.it/ ) for all enrolled students. In order to have access to this platform, students must use their username and password. Additional materials useful for the preparation of the exam will be made available on the platform IOL.
Additional useful readings
-
B. Everitt, T. Hothorn, An introduction to applied multivariate analysis with R. Springer, 2011. Chapters 1, 3, 5, 6.
-
W. K. Hardle, Z. Hlavka, Multivariate statistics, exercises and solutions. Second edition. Springer, 2015, Chapters 11, 12, 13, 14.
-
R. Johnson, D. Wichern, Applied multivariate statistical analysis. Sixth edition. Pearson, 2014. Chapters 8, 9, 11, 12.
Teaching methods
Module 1: Survey Sampling
Lectures and exercises
Module 2: Data Analysis
Theoretical lessons in a lecture hall and practical lessons in a computer laboratory.
Each statistical multivariate method listed in the course contents is described (in a lecture hall) starting from its main theoretical features and properties. Then, the corresponding functions and scripts in the R environment are detailed together with some illustrative examples (in a computer laboratory).
Although attending lessons is not mandatory, it is strongly recommended.
Assessment methods
The assessment aims to evaluate the achievement of the following learning objectives:
-
knowledge of the fundamental aspects of sampling from finite populations;
-
ability to properly use the statistical tools for design sampling plans;
-
knowledge of the multivariate analysis methods explained in the lectures;
-
ability to properly use the explained multivariate methods to analyse data matrices.
The exam is written. The overall evaluation is expressed as a grade out of 30 and is the average of the evaluations of the two units.
The exam of the Survey Sampling lasts on huor and takes place in a room. It is composed of exercises and some open questions. During the exam it is allowed the use of a form (maximun a protocol sheet), while it is not allowed to use textbooks or notes. A pocket calulator is necessary.
The exam of the Data analysis unit lasts one hour and takes place in a room. It is composed of four exercises with open questions: some concern the theoretical aspects of the multivariate statistical methods, some other are mainly focused on the ability to use methods for data analysis and interpret results from their application. These latter questions require solving numerical exercises. In some cases, results obtained from the analysis of a real data set through the R packages illustrated during the lessons may be provided. Consulting textbooks or notes during the exam is not allowed. A pocket calculator is necessary. The maximum mark for each exercise is 8. The overall mark of the Data analysis unit is given by the sum of the marks in the four exercises, which is expressed on a scale of 30.
Further useful information about the exam
-
In order to take the exam, students are required to put their names down for the exam through Almaesami platform. An identity card is required to take part in the exam.
-
Exams can only be taken in the official exam sessions.
-
Exams of the two units can be taken in different exam sessions.
Teaching tools
Slides.
Links to further information
https://corsi.unibo.it/laurea/ScienzeStatistiche
Office hours
See the website of Michele Scagliarini
See the website of Gabriele Soffritti
SDGs
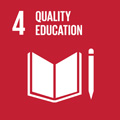
This teaching activity contributes to the achievement of the Sustainable Development Goals of the UN 2030 Agenda.