- Docente: Margherita Fort
- Credits: 6
- SSD: SECS-P/05
- Language: English
- Teaching Mode: Traditional lectures
- Campus: Bologna
- Corso: Second cycle degree programme (LM) in Economics (cod. 8408)
Learning outcomes
The course illustrates the most recent identification strategies for the quantitative assessment of causal effects using observational data by referring to micro-econometric applications. It will cover matching and difference-in-differences strategies, and quasi-experimental approaches to identification. At the end of the class, student will be able: - to critically understand the application of these tools in the recent empirical economic literature; - to apply these approaches to design his/her own program evaluation.
Course contents
The course covers empirical strategies for applied (mainly micro-economics) research questions. The main goal of the course is to provide an overview of the statistical tools for counterfactual analysis. The course illustrates the identification strategies, estimation and other related issues (eg internal and external validity) that are relevant for the assessment of causal effects (or equivalently treatment effects) using observational data. Specifically it will cover an introduction to a set of basic tools: matching and difference-in-difference methods, quasi-experimental/natural experiments settings. It will also briefly touch on approaches that allow for heterogeneous treatment effects and do not assume additive heterogeneity.
Fixed effects and random effects model are covered in other courses offered within the program and will not be discussed in detail. We will touch on fixed effects models, because they are related to the Difference-in-Differences approach to identification (and this is a pre-requisite to discuss Synthetic Control approach, and Difference-in-Discontinuities).
We will cover Instrumental Variable Strategies in detail as they are pre-requisite to discuss Fuzzy-Regression Discontinuity. Please note that, compared to standard econometrics courses, we will discuss advanced issues related to IV (LATE interpretation, identification of moments beyond averages, external validity).
We will discuss Randomized Trials because they represent the benchmark of non-experimental methods. Experimental methods are illustrated and discussed in detail in other courses at graduate and undergraduate level.
Features of each particular econometric tool will be illustrated from both the theoretical and practical point of view, often through the discussion of empirical applications.
The emphasis will be on the practical implementation of each approach.
Topics
- Fundamentals of Impact Evaluation (approximately 9 hours, including practice sessions)
- The Fundamental Problem of Causal Inference
- Potential Outcomes Framework
- Basic Approaches to Identification:Randomized Trials
- Basic Approaches to Identification:Selection on Observables
- Basic Approaches to Identification: Panel Data/Repeated Cross Section Strategies: Difference-in-Differences
- Synthetic Control Methods
- Quasi-Experiments: IV(approximately 15 hours, including practice sessions)
- Instrumental Variables: Identification, Estimation, Falsification Checks (Placebo), Interpretation (LATE), External Validity; Weak Instruments; (Fuzzy Difference-in-Differences)
- Quantile Regression (QR) for Impact Evaluation: QR with exogenous regressors; IV strategies with quantile regression: The LATE-QTE model (Abadie et al. 2002); Causal Chain Model (Chesher, 2003); The IV-QTE Model (Chernozhucov et al. 2005)
- Quasi-Experiments: Jumps and Kinks (approximately 6 hours, including practice session)
- Regression Discontinuity Design: Sharp and Fuzzy Designs, Identification, Estimation, Falsification Checks
- Multiple Cutoffs, Multiple Running Variables (eg. Geographic RDD)
- External Validity: Extrapolating Away from the Cutoff
- Regression Kink Designs: Overview (identification, estimation, falsification checks)
Readings/Bibliography
Lecture will be based on the following books and articles. We will rely on many empirical applications, referring to the recent literature. The list of papers with empirical applications will be distributed at the beginning of the class.
BOOKS
Mostly Harmless Econometrics, Angrist and Pischke
Mastering 'Metrics: The Path from Cause to Effect, Angrist and Pischke
Le lezioni riguarderanno materiale ripreso dalle fonti elencate in seguito. Gli articoli relativi alle applicazioni empiriche si riferiranno alla letteratura più recente e la lista verrà fornita all'inizio del corso.
LIBRI
Mostly Harmless Econometrics, Angrist and Pischke
Mastering 'Metrics: The Path from Cause to Effect, Angrist and Pischke
ARTICOLI
TOPIC 1: FUNDAMENTALS OF IMPACT EVALUATION
Potential outcomes, experimental benchmark, selection on observed an unobserved variables
• Abadie, A. and Cattaneo, M. (2017) Econometric Methods for Program Evaluation mimeo available at: https://economics.mit.edu/files/13924
• Holland, Paul W (1986) Statistics and Causal Inference, Journal of the American Statistical Association 81 (396): pp. 945-970, with discussion
Difference-in-Differences
• Athey and Imbens (2006) Identification and Estimation in Nonlinear Difference-in-Differences Models, Econometrica 74(2) pp.431-497
• Betrand et al. (2004) How Much Should We Trust Difference-in-Differences Estimates?, Quarterly Journal of Economics 119, pp. 245-279
• Card, D and Krueger, A. (1994) Minimum Wages and Employment: A Case Study of the Fast-Food Industry in New Jersey and Pennsylvania, American Economic Review 84(4), pp. 772-793
Computing Standard Errors
• Abadie, A. and Athey, S. and Imbens, G. (2017) When Should You Adjust Standard Errors for Clustering? WP October 2017
Propensity Score Matching
• Dehejia, R.H. and Wahba, S. (1999) Causal Effects in Non-Experimental Studies: Re-evaluating the Evaluation of Training Programs, Journal of the American Statistical Association 94 (448) pp. 1053-1062
• Dehejia, R.H. and Wahba, S. (2002) Propensity Score-Matching Methods for Nonexperimental Causal Studies, Review of Economics and Statistics, 84(1), pp. 151-161
• Dehejia, R.H. and Wahba, S. (2005) Practical Propensity Score Matching. A Reply to Smith and Todd, Journal of Econometrics, 125, pp. 355-364.
• Rosenbaum, P.R. and Rubin, D.B. (1983) The Central Role of the Propensity Score in Observational Studies for Causal Effects, Biometrika 70(1), pp.41-55
• Rosenbaum, P.R. and Rubin, D.B. (1984) Reducing Bias in Observational Studies using Subclassification on the Propensity Score, Journal of the American Statistical Association 79 (387), pp. 147-156
• Smith, J. and Todd, P. (2005a) Does Matching Overcome Lalonde’s critique of Non-Experimental Estimators? Journal of Econometrics 125, pp. 305-353
• Smith, J. and Todd, P. (2005b) Rejonder Journal of Econometrics 125, pp. 365-375
Synthetic Control Methods
• Abadie, A. and Diamond, A. and Hainmueller, J. (2015) Comparative Politics and the Synthetic Control Method, American Journal of Political Science, 59(2), pp. 495-510
• Abadie, A. and Diamond, A. and Hainmueller, J. (2010) Synthetic Control Methods for Comparative Case Studies: Estimating the Effect of California’s Tobacco Control Program, Journal of the American Statistical Association, 105(490), pp. 493-505.
394-410 TOPIC 2: QUASI-EXPERIMENTS: IV
Instrumental Variables
• Angrist, J., Imbens, G. and Rubin, D. (1996) Identification of Causal Effects Using Instrumental Variables, Journal of the American Statistical Association, 91 (434) pp. 444-455, with discussion
• Angrist, J. and Graddy, K. and Imbens G. (2000) The Interpretation of Instrumental Variables Estimators in Simultaneous Equations Models with and Application to the Demand For Fish, Review of Economic Studies, 67 pp. 499-527
• Angrist, J. (2004) Treatment Effect Heterogeneity in Theory and Practice, The Economic Journal, 114 (494) p.C52-C83
• Angrist,and Fernandez-Val (2013) ExtrapoLATE-ing: External Validity and Overidentification in the LATE Framework, in Advances in Econometric Theory and Applications, 10th World Congress, Volume III
• Imbens, G. and Rubin, D. (1997) Estimating the Outcome Distribution for Compliers in Instrumental Variables Models, Review of Economic Studies, 64, pp. 555-574
• Bound, J. and Jaeger, D. and Baker, R. (1995) Problems with Instrumental Variables Estimation when the Correlation Between the Instruments and the Endogenous Variables is Weak, Journal of the American Statistical Association 90, 443-450
• Staiger and Stock (1997) Instrumental Variable Regressions with Weak Instruments, Econometrica 65 (3), pp. 557-586
• Yogo, M., Wright, J, and Stock (2002) A Survey of Weak Instruments and Weak Identification in Generalized Method of Moments, Journal of Business and Economic Statistics 20, pp. 518-529
Quantile regression
• Abadie, A. et al. (2002) Instrumental Variable Estimates of the Effect of Subsidized Training on the Quantiles of Trainee Earnings, Econometrica, Vol. 70 (1), pp. 91-117.
• Bitler, M. et al. (2006 What Mean Impacts Miss: Distributional Effects of Welfare Reforms Experiments, American Economic Review, 96 (4) pp. 988-1012
• Chernozhucov, V. et al. (2004) The Effects of 401(K) Participation on the Wealth Distribution: An Instrumental Quantile Regression Analysis, The Review of Economics and Statistics, Vol. 86 (3), pp. 735-751.
• Chernozhucov, V. et al. (2005) An IV Model of Quantile Treatment Effects, Econometrica, Vol. 73 (1), pp. 245-261.
• Chernozhucov, V. et al. (2006) Instrumental Quantile Regression Inference for Structural and Treatment Effect Models, Journal of Econometrics
• Chesher, A. (2003) Identification in Nonseparable Models, Econometrica, Vol. 71, pp. 1405-1441
• Heckman, J.J. and Smith, J. and Clements, N. (1997) Making the Most Out of Programme Evaluations and Social Experiments: Accounting for Heterogeneity in Programme Impacts, Review of Economic Studies, 64 (4), pp. 487-535
• Imbens, G. and Rubin, D. (1997) Estimating the Outcome Distribution for Compliers in Instrumental Variables Models, Review of Economic Studies, 64, pp. 555-574
• Koenker, R. and Hallock, K.F. (2002) Quantile Regression, Journal of Economic Perspectives 15(4) pp. 143-156
• Koenker, R. (2005) Quantile Regression, Cambridge University Press, Econometric Society Monograph 38
• Ma, L. et al (2006) Quantile Regression Methods for Recursive Structural Equation Models, Journal of Econometrics, 134(2), pp. 471-506
TOPIC 3 QUASI-EXPERIMENTS: JUMPS AND KINKS
Regression Discontinuity Design
• Angrist and Lavy (1999) Using the Maimonides Rule to Estimate the Effect of Class Size on Scholastic Achievement, Quarterly Journal of Economics
• Cook (2008) Waiting for Life to Arrive: A History of the Regression-Discontinuity Design in Psychology, Statistics and Economics, Journal of Econometrics 142, pp.636-654
• Hahn, J. and Todd, P. and Van der Klaauw, W. (2001) Identification and Estimation of Treatment Effects with a Regression Discontinuity Design, Econometrica 69 (1)
• Imbens, G. and Lemieux, T. (2008) Regression Discontinuity Designs: A Guide to Practice, Journal of Econometrics 142 (2), pp. 615-635 and papers on the same journal in the special issue The Regression Discontinuity Design: Theory and Applications, Journal of Econometrics 142(2), pp. 611-850
• Research work by Cattaneo and co-authors https://sites.google.com/site/matiasdcattaneo/
RD away from the cutoffs (external validity); multiple cutoffs; multiple running variables
• Angrist, J. and Rokkanen, M. (2015) Wanna Get Away? Regression Discontinuity Estimation of Exam School Effects Away from the Cutoff, Journal of the American Statistical Association
Teaching methods
Each topic will be covered in class and in a computer laboratory practice session.
The lessons will cover the presentation of theoretical and applied topics related to the various econometric tools. The applications will be illustrated in the classroom and then resumed in practical lessons in the computer lab using STATA.
All teaching materials will be distributed through the e-learning platform of the University of Bologna.
Research articles listed among the references can be downloaded from the web. You may use the search engine: http://acnp.unibo.it/cgi-ser/start/it/cnr/fp.html
Most books in the reference list are available at the University libraries. You may check availability through the search engine http://sol.unibo.it/SebinaOpac/Opac?sysb =
To address the need to offer the whole course online, to make students' experience more interactive, I will experimentally adopt innovative teaching tools (such as peer instruction; see link with reference) using adequate techical support during lectures relying for instance on free available software such as Pingo (https://pingo.coactum.de/). Students do not need to install the software ahead but need to have a device (mobile phone or laptop) who can access internet during the lectures in which this approach will be implemented.
In addition, peer education requires a great deal of investment from students as students have to read the textbook before coming to class.
Assessment methods
Due the Covid-19 pandemic, exams in January and February 2021 will be online.
The assessment will be based on
- replication study (70%; max 23 out of 33 points)
Students will have to submit: a) a STATA code to replicate the results of a published paper that uses at least one of the tools illustrate in the course ; b) a Fact checking report, summarizing the results of the replciation study (guidelines to be illustrated in class); c) slides on an extension of the replicated study (eg : same analisi on more recent data/on data on another country; different analysis -with the tools illustrated in this class- on the same data)
- in class participation (20%; max 7 out of 33 points)
- questions on the course program during the oral exam/replication study audit (10% max 4 out of 33 points)
Teaching tools
Slides, teaching material , practice in the lab.
Self-evaluation on-line tests will be made available through the e-learning platform https://elearning-cds.unibo.it/
Lectures involve the presentation of theoretical and applied issues of the various econometric methods. Applications are discussed in class and replicated during the computer laboratory session using STATA.
Software STATA: available for students of the Department of Economics (CAMPUS license) and at the Computer Lab of the School of Economics and Management.
Links to further information
https://www.kuleuven.be/english/education/teaching-tips/activating-students/peer-instruction)
Office hours
See the website of Margherita Fort
SDGs
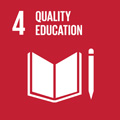
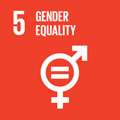
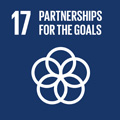
This teaching activity contributes to the achievement of the Sustainable Development Goals of the UN 2030 Agenda.