- Docente: Mariagiulia Matteucci
- Credits: 8
- SSD: SECS-S/01
- Language: Italian
- Teaching Mode: Traditional lectures
- Campus: Rimini
- Corso: First cycle degree programme (L) in Finance, Insurance and Business (cod. 8872)
Learning outcomes
At the end of the course, students know the basic concepts of statistical methodologies for taking business and financial decisions, and models for accounting and financial data for credit scoring.
Course contents
- Introduction to statistical methods for credit scoring, purposes and phases.
- Recall of categorical variables: marginal and conditional independence, measures of association.
- Logistic regression model: estimation and interpretation of model parameters, variable selection, goodness of fit.
- Discriminant analysis: canonical discriminant analysis, model-based discriminant analysis.
- Classification trees: model building and evaluation of results.
- Estimation of the classification error rate.
- Basics of neural networks.
- Basics of latent variable models.
- Recall of cluster analysis.
- Case studies.
Readings/Bibliography
Elena Stanghellini (2009) “Introduzione ai metodi statistici per il credit scoring”, Springer-Verlag
Stefania Mignani, Angela Montanari (1997) “Appunti di analisi statistica multivariata”, Esculapio (chap. 5 discriminant analysis, cap. 7 cluster analysis)
Sergio Zani, Andrea Cerioli (2007) “Analisi dei dati e data mining per le decisioni aziendali”, Giuffrè Editore (cap. 8 distances and similarity indexes, cap. 9 cluster analysis, cap. 11 classification trees, cap. 12 neural networks)
Teaching methods
Theoretical lessons and practical activities in computer laboratory with R. During the lectures, groups of students will be asked to participate actively in the discussion of case studies.
Assessment methods
Final oral test to assess the knowledge of the methodologies, including discussion of case studies with the software R. It will be possible to take two partial tests (a first written test at the end of the first period of lectures and a second oral test). The first written test consists of open-ended items dealing with both the statistical theory and the interpretation of R outputs. Prerequisites: mathematics, statistics, probability, inference.
Teaching tools
Slides, dataset, software R.
Office hours
See the website of Mariagiulia Matteucci
SDGs
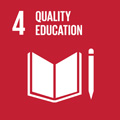
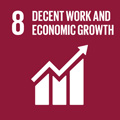
This teaching activity contributes to the achievement of the Sustainable Development Goals of the UN 2030 Agenda.