- Docente: Alessandra Luati
- Credits: 6
- SSD: SECS-S/01
- Language: English
- Teaching Mode: Blended Learning
- Campus: Bologna
- Corso: Second cycle degree programme (LM) in Statistical Sciences (cod. 9222)
-
from Apr 07, 2025 to May 09, 2025
Learning outcomes
By the end of the course the student is able to analyse data generated by GARCH, DCS, long memory processes and make inference on the moment estimators.
Course contents
Prerequisites: linear time series analysis (linear processes, Wold representation, autocovariance and spectral density function, inference on the moments of a linear process) and basic stochastic processes (conditioning, martingales, martingale difference sequences).
Topics covered throughout the course: Time varying parameter models. Parameter driven models: state space models and the Kalman filter. Observation driven models: GARCH processes and Score driven models. Estimation and inference.
Optional, additional topics: dynamic quantiles, forecast densities, invertibility, long memory processes, locally stationary processes.
Readings/Bibliography
Brockwell P.J. and Davis R.A. (1991), Time series: Theory and Methods, Springer.
Further readings will be suggested during the course.
Teaching methods
Recorded lectures, lectures and discussions in class exercises and lab sessions (R, Matlab or Python).
Assessment methods
1) written exam
2) analysis of a case study (coursework, take home)
Teaching tools
Textbook, notes and papers that can be found on the institutional teacher web-site and in Virtuale
Office hours
See the website of Alessandra Luati
SDGs
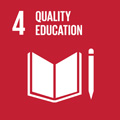
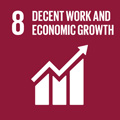
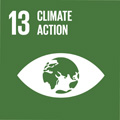
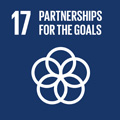
This teaching activity contributes to the achievement of the Sustainable Development Goals of the UN 2030 Agenda.