- Docente: Anna Gloria Billè
- Credits: 6
- SSD: SECS-S/03
- Language: Italian
- Teaching Mode: Traditional lectures
- Campus: Bologna
- Corso: Second cycle degree programme (LM) in Business Administration (cod. 0897)
-
from Feb 12, 2024 to Mar 15, 2024
Learning outcomes
This course will present multivariate statistical methods in several ways: (i) It offers an overview of the main multivariate methods in economics and business, especially for microdata, (ii) It helps in choosing the best statistical method for different economic data sets, (iii) A brief explanation of some advanced methods, like spatial modeling, are also included, (iv) The use of the software R will help in estimating models, testing, and displaying related graphs.
Course contents
Introduction to data: definition, types and sources, microdata, main issues related to observational data.
Linear models: intro, Gauss-Markov hypotheses and inference, Monte Carlo simulations (an example), marginal effects, dummy variables, model selection, LASSO, economic data examples. Residual analysis and specification tests, violation of the hypoteses and alternative estimators, endogeneity test, exogeneity test, endogeneity example.
Time series: intro, residual analysis and specification tests, time series components, forecasting with classical methods, missing data imputation with moving average.
Discrimination, classification and clustering: intro, multinomial logistic regression, binary probit/logit models, spatial probit model, regression trees, k-means clustering, spatial clustering.
Further topics: nonlinear models in regressors, power transformations, intro on panel data.
R programming
Readings/Bibliography
References:
Tsai Chun-Wei et al. (2015), Big Data Analytics: a survey, Journal of Big Data, 2:21.
Daniel Zelterman (2014), Applied Multivariate Statistics with
R, Springer.
Marno Verbeek (2005), Econometria, I edizione, Zanichelli
Editore.
William Greene (2019), Econometric Analysis, Pearson. Eighth
Edition (Global Edition).
Gareth James, Daniela Witten, Trevor Hastie, Robert Tibshirani (2021), An Introduction to Statistical Learning with Applications in R, Springer.
Teaching methods
Lessons are carried out considering both methodological and empirical aspects in economics, with the help of the statistical software R.
The used economic datasets are all available in R or provided by the Professor.
Assessment methods
Oral examination.
The examination consists into the evaluation of a work group.
The students are divided into groups and they will prepare a short thesis explaining the type of dataset used, the statistical method/s and the results with the use of R.
Each group will also prepare a short presentation/seminar during which some questions are made.
Teaching tools
Pc; videoprojector.
Office hours
See the website of Anna Gloria Billè
SDGs
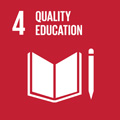
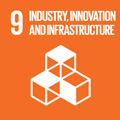
This teaching activity contributes to the achievement of the Sustainable Development Goals of the UN 2030 Agenda.