- Docente: Giuliano Galimberti
- Credits: 10
- SSD: SECS-S/01
- Language: English
- Teaching Mode: Traditional lectures
- Campus: Bologna
- Corso: Second cycle degree programme (LM) in Statistical Sciences (cod. 9222)
-
from Feb 14, 2023 to May 23, 2023
Learning outcomes
By the end of the course the student learns the basic notions to define statistical models. In particular, the student is able to estimate parameters, test hypothesis about them and build confidence intervals for generalized linear models and for linear mixed models, and to choose the most suitable model for the specific problem at hand.
Course contents
Statistical models: introduction.
Linear regression models:
- Basic definition;
- Maximum likelihood estimators;
- Hypothesis teting;
- Goodness of fit criteria;
- Model comparisons;
- extentions to allow non-linear effects.
Generalized linear models:
- Exponential families, linear predictor, link functions;
- Maximum likelihood estimators;
- Goodness of fit: the deviance of a model;
- Residual analysis;
- Inference on the parameters: likelihood ratio and Wald statistics;
- Poisson regression for count data;
- Logistic regression for categorical data;
- Other examples.
Increasing flexibility in regression models:
- Polynomial regression;
- Use of spline functions: regression splines, smoothing splines and penalized splines;
- Introduction to generalized additive models.
Readings/Bibliography
Recommended readings (a detailed list of selected chapters and sections is available on virtuale.unibo.it):
- Dobson, A. J. (2002) An Introduction to Generalized Linear Models. Second Edition. Chapman & Hall/CRC.
- Everitt, B. S., Hothorn, T. (2009) A handbook of statistical analysis using R. Second edition. Chapman & Hall/CRC.
Handouts provided by the teacher.
Other readings:
- Azzalini, A. (1996) Statistical inference based on the likelihood. Chapman & Hall/CRC.
- Agresti, A. (2015) Foundations of linear and generalized linear models. Wiley.
Teaching methods
Class lectures
Tutorial sessions in computer lab
As concerns the teaching methods of this course unit, all students must attend Module 1 and 2 on Health and Safety online
Assessment methods
The exam will test the qualifications of each student on both a theoretical and a practical level.
The exam is based on a (possibly computer-based) quiz consisting of a set of multiple-choice and open-answer questions concerning the models presented during the course. These questions focus both on theoretical properties and on output produced using the software R. As far as the multiple-choice questions, correct answers are marked with 1 point, wrong answers are marked -0.20 points and missing answers 0 points. Each open-answer question receives a mark ranging between 0 and 2, depending on the correctness of the answer and the appropriateness of the terminology. The maximum time allowed to complete the quiz is 80 minutes. The total number of multiple-choice and open-answer questions may vary from sitting to sitting, and the final marks are always rescaled on a 0-32 range. Non-integer final marks are rounded down to the next small integer. Final marks larger that 30 are rounded down to 30. Final marks equal to 32 are considered 30 cum laude.
Students are allowed to reject a positive mark and retake the exam in one of the following sittings at least once but no more than twice.
Consulting textbooks or personal notes during the exam is not allowed.
As far as the first sitting is concerned, students have the option of splitting the exam into two partial exams (maximum time for each partial exam: 40 minutes). The first partial exam takes place after the first 5 weeks and is focused is focused on the topics covered during the first part of the course (the maximum mark for the first partial exam is set to 16). The second partial written exam is scheluled after the end of the course, and it covers the topics addressed during the second part of the course (the maximum mark for the second partial exam is set to 16). Students must take both partial written exams. In particular, in order to register for the second partial exam, a student must have taken the first partial exam. In case of failure or rejection of the total mark obtained at the end of the second partial exam, students must repeat the whole written exam in one of the following sittings.
Office hours
See the website of Giuliano Galimberti
SDGs
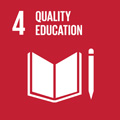
This teaching activity contributes to the achievement of the Sustainable Development Goals of the UN 2030 Agenda.